Addressing Challenges In Automated Visual Inspection Of Lyophilized Vials
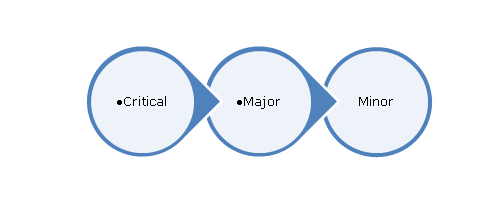
Table of Contents
Challenges Related to Image Acquisition and Preprocessing
High-quality image acquisition is fundamental to successful automated visual inspection of lyophilized vials. However, several factors can compromise image quality, leading to inaccurate inspection results.
Illumination and Shadowing
Inconsistencies in lighting are a major source of error. Non-uniform illumination can create shadows that obscure defects like cracks or particulate matter, leading to false negatives. The reflective surfaces of glass vials further complicate consistent illumination, causing glare and reflections that interfere with image analysis.
- Non-uniform illumination: Masks cracks or particulate matter.
- Reflective vial surfaces: Cause glare and hinder defect detection.
- Mitigation strategies: Advanced lighting techniques like structured illumination, coaxial lighting, or multi-spectral imaging can significantly mitigate these issues by providing more uniform and controlled illumination.
Variability in Vial Appearance
Variations in vial appearance introduce significant challenges for automated visual inspection systems. Differences in vial color, fill level, and stopper positioning all impact the effectiveness of image analysis algorithms. Furthermore, the lyophilized cake itself can exhibit considerable variability in color, texture, and appearance, making it difficult to distinguish between acceptable variations and actual defects.
- Lyophilized cake variability: Differences in color, texture, and structure can be misinterpreted as defects.
- Fill level inconsistencies: Varying fill levels can affect the appearance of the cake and introduce shadowing.
- Solutions: Machine learning algorithms trained on extensive and diverse datasets are crucial to handle this variability. Pre-processing techniques, such as image normalization and standardization, can help reduce the impact of these variations.
High-Throughput Demands
The pharmaceutical industry demands high-throughput inspection of large batches of lyophilized vials. Balancing the need for speed with the requirement for high accuracy poses a significant challenge.
- Speed vs. accuracy: Efficient algorithms and hardware are necessary to achieve both high speed and high accuracy.
- System integration: Seamless integration with high-speed conveyor systems is critical for efficient processing.
- Parallel processing: Implementing parallel processing and distributed computing can dramatically increase throughput.
Challenges Related to Defect Detection and Classification
Accurate and reliable defect detection and classification are crucial for ensuring the quality of lyophilized vials. However, several factors make this a complex task.
Subtle Defect Identification
Detecting small cracks, microscopic particulate matter, or subtle variations in the lyophilized cake requires advanced image processing techniques. These defects are often difficult to identify visually, even by trained inspectors.
- Advanced image processing: Techniques like edge detection, texture analysis, and wavelet transforms can enhance the visibility of subtle defects.
- Machine learning: Deep learning models, in particular, are proving highly effective at detecting subtle defects that might be missed by traditional methods.
- Model retraining: Regular retraining of machine learning models with diverse and updated defect datasets is crucial for maintaining consistent performance.
False Positives and False Negatives
Minimizing false positives (incorrectly identifying a non-defect as a defect) and false negatives (missing actual defects) is critical. A balance between sensitivity (detecting all defects) and specificity (avoiding false positives) must be carefully managed.
- Algorithm design: Careful design and parameter tuning of algorithms are crucial for minimizing misclassifications.
- Statistical analysis: Robust statistical analysis of performance metrics (precision, recall, F1-score) is necessary to evaluate the system's accuracy.
- Quality control: Implementation of quality control checks and human oversight, especially for initially flagged defects, can further reduce errors.
Challenges Related to System Integration and Validation
Successful implementation of automated visual inspection systems requires careful planning and validation to ensure seamless integration with existing production lines.
Integration with Existing Production Lines
Integrating automated systems into existing filling and packaging lines requires careful consideration of compatibility with different vial types and sizes, along with data integration with existing manufacturing execution systems (MES).
- Compatibility: The system must be compatible with a wide range of vial types and sizes to handle variations in production.
- Data integration: Seamless data exchange with MES is critical for efficient tracking and management of inspection results.
- Regulatory compliance: Validation according to relevant regulatory guidelines, such as FDA 21 CFR Part 11, is essential to ensure compliance.
Data Management and Analysis
Automated visual inspection systems generate vast amounts of data. Efficient management, analysis, and storage of this data are crucial for optimizing system performance and ensuring data integrity.
- Data storage: Secure and robust data storage and retrieval systems are necessary.
- Data analytics: Data analytics tools can help identify trends, optimize inspection parameters, and improve overall system performance.
- Data integrity: Compliance with data integrity regulations is paramount to ensure the reliability and trustworthiness of inspection results.
Conclusion
Automated visual inspection of lyophilized vials offers significant advantages in terms of efficiency and accuracy compared to manual inspection. However, several challenges related to image acquisition, defect detection, and system integration need to be addressed. By implementing advanced imaging techniques, robust machine learning algorithms, and careful system validation, the pharmaceutical industry can overcome these hurdles and realize the full potential of automated visual inspection. Investing in and optimizing automated visual inspection systems is crucial for ensuring the consistent quality and safety of lyophilized pharmaceuticals. Embrace the future of quality control with advanced solutions for automated visual inspection of lyophilized vials.
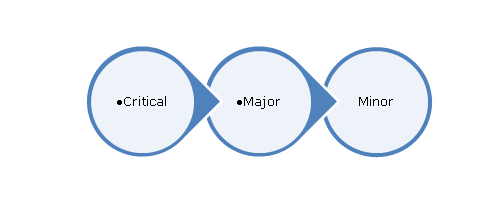
Featured Posts
-
Who Will Be The Next Pope Analyzing The Leading Contenders
May 11, 2025 -
Henry Golding On Crazy Rich Asians Reunions And The Upcoming Tv Series
May 11, 2025 -
The Chaplin Factor Key To Ipswich Towns Wins
May 11, 2025 -
Prints Endryu 65 Rokiv Ditinstvo U Fotografiyakh
May 11, 2025 -
Verbesserung Der Asylunterkuenfte Einsparungspotenzial Von 1 Milliarde Euro
May 11, 2025