AI's Cognitive Processes: A Deep Dive Into Its Functionality
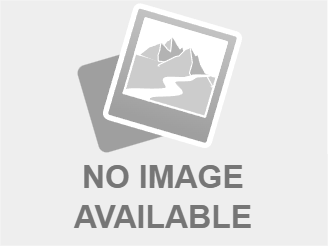
Table of Contents
Learning Mechanisms in AI's Cognitive Processes
AI's ability to learn is a cornerstone of its cognitive capabilities. This learning is achieved through various methods, broadly categorized as supervised, unsupervised, and reinforcement learning. Understanding these learning mechanisms is crucial to grasping the full spectrum of AI's cognitive functions.
Supervised Learning
Supervised learning involves training an AI model on a large dataset of labeled data. This means each data point is tagged with the correct answer or classification. The algorithm learns to map inputs to outputs based on this labeled data.
- Process: The algorithm analyzes the labeled data, identifying patterns and relationships between the input features and the corresponding outputs. It then creates a model that can predict the output for new, unseen inputs.
- Algorithms: Common algorithms used in supervised learning include Support Vector Machines (SVMs), Logistic Regression, and various types of decision trees.
- Examples: Image recognition (classifying images as cats or dogs), spam filtering (identifying emails as spam or not spam), and medical diagnosis (predicting diseases based on patient data).
- Advantages: High accuracy on well-defined problems with sufficient labeled data.
- Limitations: Requires large amounts of labeled data, which can be expensive and time-consuming to obtain. It may struggle with noisy or incomplete data.
Unsupervised Learning
Unsupervised learning tackles unlabeled data – data without predefined categories or outputs. The AI's task is to identify patterns, structures, and relationships within the data itself.
- Process: The algorithm analyzes the data to discover inherent structures and patterns, grouping similar data points together or identifying anomalies.
- Algorithms: Clustering algorithms like k-means and DBSCAN group similar data points. Dimensionality reduction techniques like Principal Component Analysis (PCA) reduce the number of variables while preserving important information.
- Examples: Customer segmentation (grouping customers based on purchasing behavior), anomaly detection (identifying fraudulent transactions), and topic modeling (discovering topics in a collection of documents).
- Advantages: Can handle large amounts of unlabeled data and uncover hidden patterns.
- Limitations: The interpretation of results can be subjective and requires careful analysis.
Reinforcement Learning
Reinforcement learning involves training an AI agent to interact with an environment, learning through trial and error. The agent receives rewards for desirable actions and penalties for undesirable ones.
- Process: The agent learns an optimal policy – a strategy for selecting actions that maximize cumulative rewards over time.
- Algorithms: Q-learning, SARSA, and Deep Q-Networks (DQNs) are common reinforcement learning algorithms.
- Examples: Game playing (AlphaGo), robotics (learning to walk or manipulate objects), and resource management (optimizing energy consumption).
- Advantages: Can learn complex behaviors and adapt to changing environments.
- Limitations: Can be computationally expensive and requires careful design of the reward function to ensure desired behavior.
Decision-Making Frameworks in AI's Cognitive Processes
Once AI systems have learned from data, they need mechanisms to apply this knowledge and make decisions. Several frameworks guide this process, each with its strengths and weaknesses.
Rule-Based Systems
Rule-based systems make decisions by following pre-defined rules and logical inferences. These rules are often created by human experts.
- Process: The system receives input, matches it against its rules, and deduces a conclusion based on the rules' conditions.
- Examples: Expert systems for medical diagnosis, decision support systems for financial analysis.
- Limitations: Can be brittle and inflexible, struggling with situations not explicitly covered by the rules.
Probabilistic Reasoning
Probabilistic reasoning uses probability and statistics to make decisions under uncertainty. This is crucial when dealing with incomplete or noisy data.
- Process: The system uses probability distributions to represent uncertainty and update its beliefs based on new evidence.
- Examples: Bayesian networks for medical diagnosis, Markov models for predicting customer churn, risk assessment models in finance.
- Advantages: Can handle uncertainty and incomplete information.
- Limitations: Requires accurate probability estimations, which can be challenging to obtain.
Neural Networks and Decision-Making
Neural networks, particularly deep learning models, are powerful tools for decision-making. They can learn complex patterns and relationships from data, enabling sophisticated decision-making capabilities.
- Process: Input data is processed through multiple layers of interconnected nodes, each performing calculations and transforming the data. The final layer produces the output, representing the decision.
- Examples: Convolutional Neural Networks (CNNs) for image recognition, Recurrent Neural Networks (RNNs) for natural language processing, and deep reinforcement learning for complex control tasks.
- Advantages: Can handle high-dimensional data and learn complex relationships.
- Limitations: Can be computationally expensive to train and may require large datasets. Their decision-making processes can be opaque ("black box").
Problem-Solving Strategies within AI's Cognitive Processes
AI employs various strategies to tackle problems, ranging from straightforward search algorithms to sophisticated heuristic approaches.
Search Algorithms
Search algorithms systematically explore possible solutions to a problem.
- Process: The algorithm explores a search space, evaluating possible solutions until a satisfactory solution is found.
- Examples: A* search for pathfinding, breadth-first search for exploring game trees, depth-first search for solving puzzles.
- Advantages: Guarantees finding a solution (for certain algorithms) if one exists.
- Limitations: Can be computationally expensive for large search spaces.
Heuristics and Optimization
Heuristics are rules of thumb that guide the search for solutions, often sacrificing optimality for efficiency. Optimization techniques aim to find the best solution among a set of possibilities.
- Process: Heuristics provide shortcuts to navigate the search space, while optimization algorithms systematically refine solutions to improve their quality.
- Examples: Genetic algorithms for optimization problems, simulated annealing for finding near-optimal solutions.
- Advantages: Can handle complex problems where exhaustive search is impractical.
- Limitations: May not always find the optimal solution.
Knowledge Representation and Reasoning
Effective problem-solving requires representing knowledge and using reasoning to deduce solutions.
- Process: Knowledge is represented using formal structures like semantic networks or ontologies. Inference engines use these representations to derive new knowledge and solve problems.
- Examples: Expert systems using rule-based reasoning, semantic search engines using knowledge graphs.
- Advantages: Allows for complex reasoning and handling of symbolic information.
- Limitations: Requires careful design of knowledge representation and inference mechanisms.
Conclusion: Understanding AI's Cognitive Processes for the Future
This article explored the core functionalities of AI's cognitive processes, covering its learning mechanisms, decision-making frameworks, and problem-solving strategies. We examined different types of learning (supervised, unsupervised, reinforcement), decision-making approaches (rule-based, probabilistic, neural networks), and problem-solving techniques (search algorithms, heuristics, knowledge representation). Advancements in AI's cognitive capabilities have profound implications across various fields, from revolutionizing healthcare through improved diagnostics and personalized medicine to optimizing financial markets and enhancing manufacturing processes. Understanding AI's cognitive functions is crucial for navigating the future. Continue your exploration of this fascinating field to fully grasp the potential and implications of artificial intelligence and its cognitive abilities. To delve deeper, explore resources on machine learning algorithms, deep learning architectures, and cognitive computing frameworks. Mastering AI's cognitive processes is key to harnessing the power of this transformative technology.
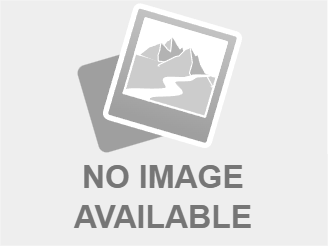
Featured Posts
-
Willie Nelson Documentary Leading Austin News
Apr 29, 2025 -
Minnesota Snow Plow Naming Contest Winners Revealed
Apr 29, 2025 -
How U S Companies Are Managing Costs Under Tariff Uncertainty
Apr 29, 2025 -
European Power Prices Plunge Solar Energy Surplus Drives Negative Prices
Apr 29, 2025 -
Understanding The Surge In The Venture Capital Secondary Market
Apr 29, 2025
Latest Posts
-
Willie Nelson Releases 77th Solo Album Ahead Of 92nd Birthday
Apr 29, 2025 -
Willie Nelsons New Documentary A Tribute To His King Of The Road Crew
Apr 29, 2025 -
Willie Nelsons 77th Solo Album A Pre 92nd Birthday Release
Apr 29, 2025 -
Willie Nelson Documentary Leading Austin News
Apr 29, 2025 -
Willie Nelson Documentary Tops Austin News This Week
Apr 29, 2025