D-Wave's QBTS: Advancing Drug Discovery Through Quantum AI
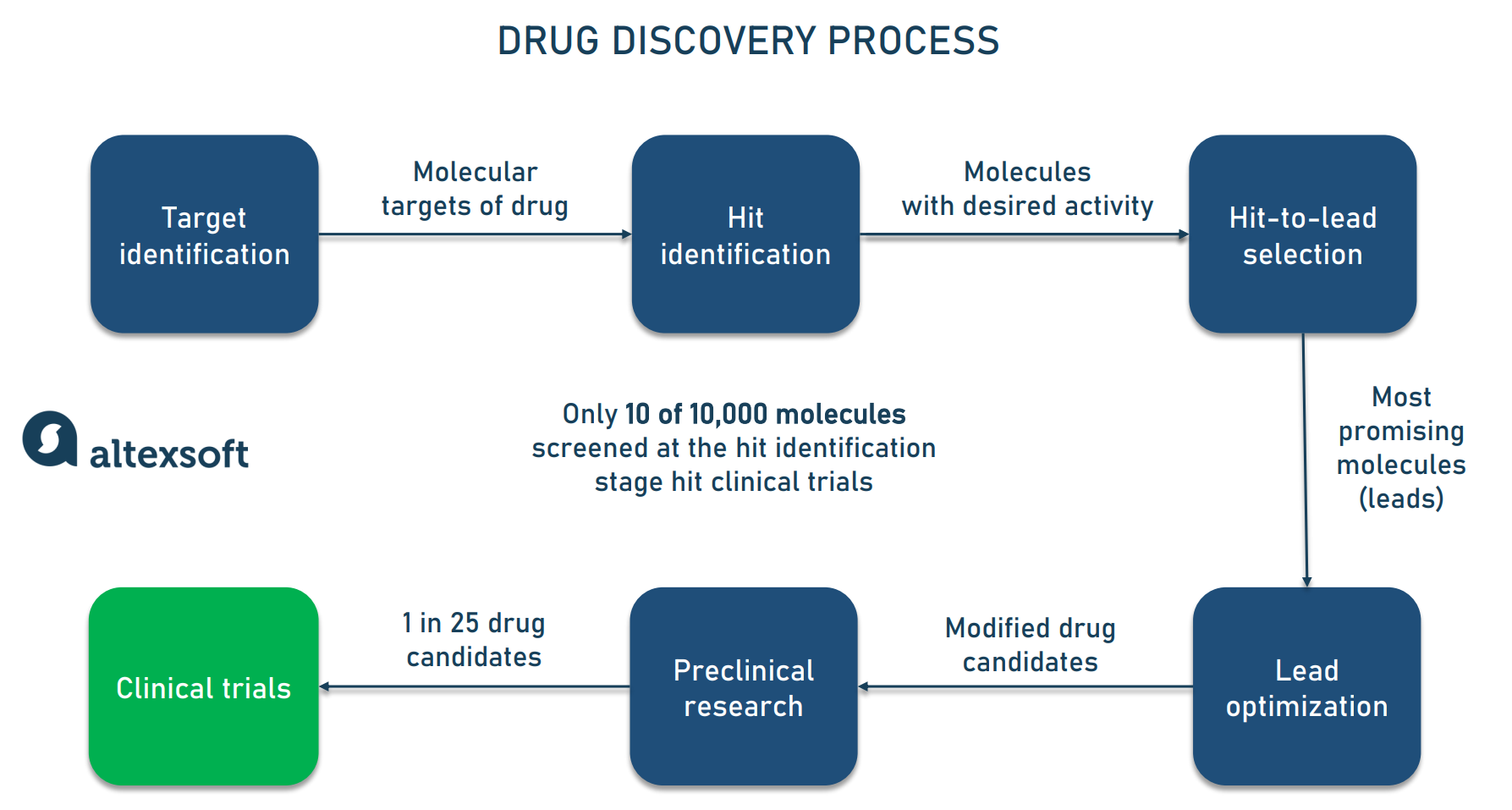
Table of Contents
Understanding D-Wave's QBTS and its Capabilities
What is Quantum Annealing?
Quantum annealing is a specialized type of quantum computing designed to solve complex optimization problems far more efficiently than classical computers. It leverages the principles of quantum mechanics to explore a vast solution space simultaneously, finding the optimal solution much faster. This speed advantage is particularly crucial in drug discovery, where researchers grapple with incredibly complex molecular simulations.
- Speed and Efficiency Gains: Quantum annealing offers exponential speedups compared to classical algorithms for certain types of problems, dramatically reducing the time required for simulations and analyses.
- Practical Application in Drug Discovery: Quantum annealing can optimize drug molecule design, predict protein folding, and analyze vast datasets of molecular interactions, accelerating the identification of promising drug candidates.
- Comparison with Other Quantum Computing Approaches: While quantum annealing is distinct from other quantum computing models like gate-based quantum computing, it excels in solving specific optimization problems relevant to drug discovery.
The Role of Quantum Boltzmann Machines (QBMs)
Quantum Boltzmann Machines (QBMs) are a type of quantum neural network ideally suited for analyzing complex datasets. They are particularly powerful in scenarios involving high-dimensional data, like those encountered in protein folding and understanding intricate molecular interactions. QBMs learn patterns and relationships from data far more effectively than their classical counterparts.
- Faster Pattern Learning: QBMs can learn patterns from massive datasets of molecular structures and interactions at a speed unattainable by classical machine learning methods.
- Identifying Drug Targets and Predicting Properties: QBMs excel at identifying potential drug targets and predicting crucial molecular properties, such as binding affinity and solubility.
- Scalability for Large Datasets: QBMs are designed to handle the enormous datasets typical in pharmaceutical research, enabling researchers to analyze far more data and uncover hidden patterns.
QBTS: A Temporal Extension for Enhanced Performance
The Quantum Boltzmann Temporal Sampler (QBTS) extends the capabilities of QBMs by adding a temporal dimension. This crucial enhancement allows for the modeling of dynamic processes—essential for understanding how drugs behave over time. This temporal perspective improves the accuracy and predictive power of the simulations significantly.
- Improved Accuracy and Prediction: QBTS provides more accurate predictions of drug efficacy and side effects by incorporating the temporal evolution of molecular interactions.
- Time-Dependent Interactions: QBTS allows researchers to model time-dependent processes, such as drug metabolism and drug-target interactions over time, leading to more realistic simulations.
- Personalized Medicine and Drug Repurposing: QBTS's ability to model dynamic systems holds immense potential for personalized medicine and drug repurposing by allowing for simulations tailored to individual patient characteristics.
QBTS Applications in Drug Discovery
Accelerating Lead Compound Identification
QBTS dramatically accelerates the identification of promising drug candidates by analyzing vast chemical libraries. It surpasses traditional high-throughput screening (HTS) methods in both speed and efficiency.
- Speed Improvement over HTS: QBTS significantly reduces the time required to screen large chemical libraries, leading to faster identification of potential drug candidates.
- Examples in Drug Discovery: QBTS can be used to identify potential inhibitors for specific diseases, such as cancer or Alzheimer's disease, by analyzing the interactions between drug molecules and target proteins.
- Cost-Effectiveness: The speed and efficiency gains translate into significant cost savings in the early stages of drug discovery.
Optimizing Drug Design and Development
QBTS helps refine drug molecules to enhance their efficacy and reduce side effects. By predicting drug-target interactions and optimizing drug delivery, it streamlines the drug design process.
- Predicting Drug-Target Interactions: QBTS accurately predicts how drug molecules interact with their target proteins, aiding in the design of more effective drugs.
- Optimizing Drug Delivery Methods: QBTS can be used to optimize drug delivery methods, such as nanoparticles or liposomes, to improve drug absorption and reduce side effects.
- Improved Pharmacokinetic Properties: QBTS contributes to designing drugs with improved pharmacokinetic properties, such as better absorption, distribution, metabolism, and excretion (ADME).
Predicting Drug Efficacy and Safety
QBTS significantly improves the accuracy of predictions regarding a drug's effectiveness and potential adverse effects. This enhanced predictive power reduces the need for extensive and costly clinical trials, leading to faster and safer drug development.
- Reducing Drug Failure Risk: By accurately predicting efficacy and safety early on, QBTS minimizes the risk of costly drug failures in later stages of development.
- Faster and Safer Drug Development: QBTS enables a faster and more efficient drug development process, bringing life-saving medications to patients more quickly.
- Reducing Healthcare Costs: The speed and efficiency gains translate into significant cost savings for the healthcare system.
The Future of QBTS and Quantum AI in Drug Discovery
Ongoing Developments and Advancements
Research into QBTS and its applications is constantly evolving. Ongoing developments promise even greater capabilities in the future.
- Algorithmic Efficiency Improvements: Continuous efforts are underway to improve the algorithmic efficiency of QBTS, leading to even faster and more accurate simulations.
- Collaboration with Pharmaceutical Companies: D-Wave actively collaborates with leading pharmaceutical companies to explore and implement QBTS in real-world drug discovery projects.
- Hybrid Quantum-Classical Algorithms: Hybrid algorithms, combining the strengths of quantum and classical computing, hold immense potential for further advancements.
The Potential for Transforming the Pharmaceutical Industry
QBTS and quantum AI hold the potential to fundamentally transform the drug discovery landscape.
- Faster Time-to-Market: QBTS can significantly shorten the time required to bring new drugs to market, accelerating access to life-saving treatments.
- More Effective and Safer Treatments: The improved prediction capabilities of QBTS lead to the development of more effective and safer drugs with fewer side effects.
- Increased Accessibility of Medications: The cost savings and efficiency gains associated with QBTS can contribute to increased accessibility of life-saving medications.
Conclusion
D-Wave's QBTS represents a significant advancement in the application of quantum AI to drug discovery. By leveraging the power of quantum annealing and Boltzmann machines, QBTS accelerates lead compound identification, optimizes drug design, and enhances prediction capabilities. This technology promises to revolutionize the pharmaceutical industry, paving the way for innovative treatments and improved healthcare outcomes. To learn more about how D-Wave's QBTS can advance your drug discovery efforts, explore the possibilities of quantum computing and the potential of quantum AI for drug discovery today.
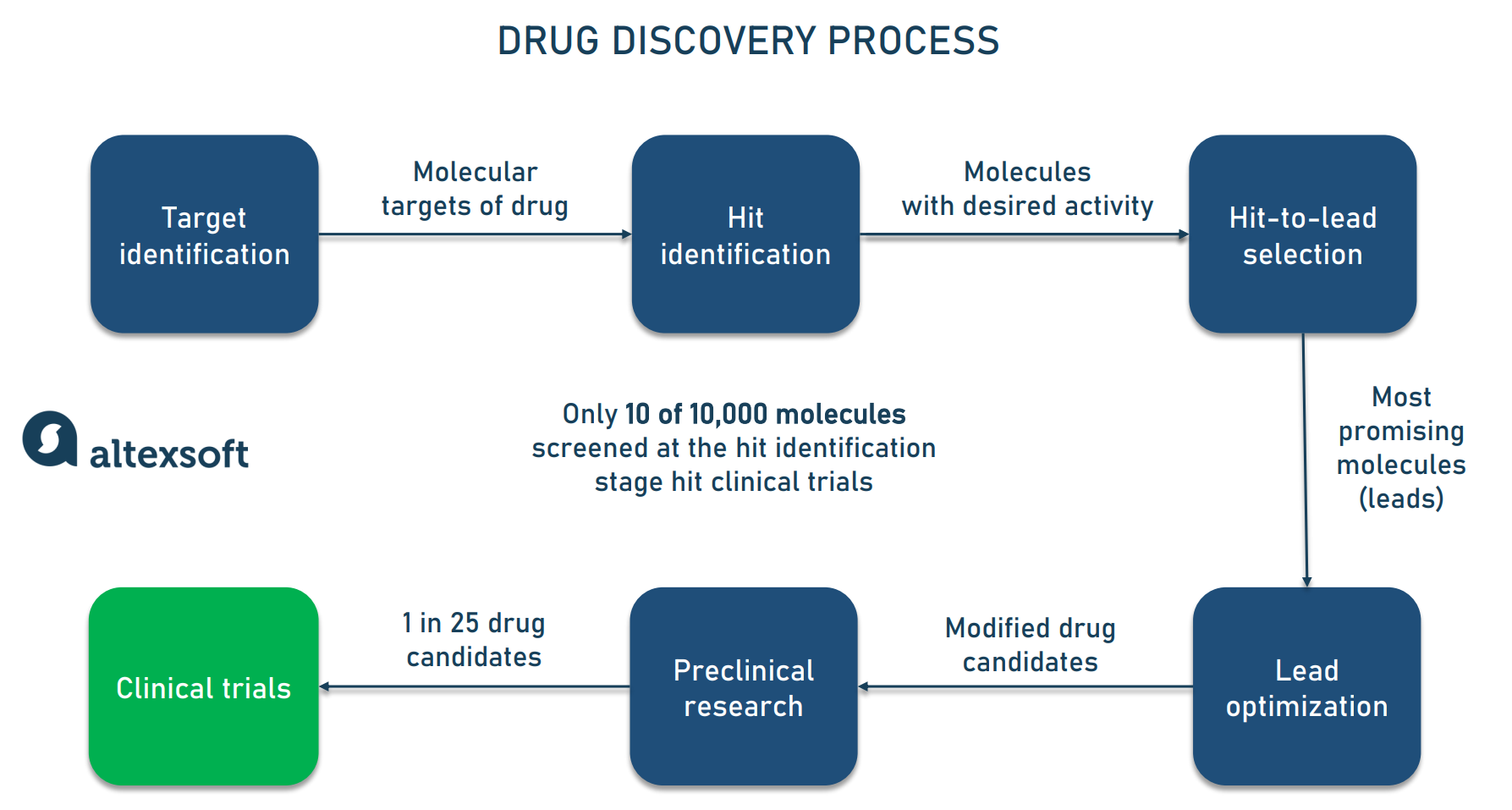
Featured Posts
-
Strike Over Nj Transit Engineers Reach Agreement With Union
May 20, 2025 -
Tottenham Loanee Leads Leeds Back To Championship Top
May 20, 2025 -
Fate Of Abc News Show Uncertain Following Mass Layoffs
May 20, 2025 -
Tonci Tadic O Pregovorima Putinov Plan I Njegovi Ciljevi
May 20, 2025 -
Ecowas Economic Affairs Strategic Planning In Niger
May 20, 2025
Latest Posts
-
David Walliams Fing Production Greenlit By Stan
May 20, 2025 -
Fing A New David Walliams Fantasy Film Coming Soon Thanks To Stan
May 20, 2025 -
Mia Wasikowska Joins Taika Waititis New Family Film
May 20, 2025 -
Stan Gives The Go Ahead To David Walliams Fing Fantasy Adaptation
May 20, 2025 -
David Walliams Fing Stans Greenlight And What To Expect
May 20, 2025