How Google Trains Its Search AI Even After Web Content Opt-Out
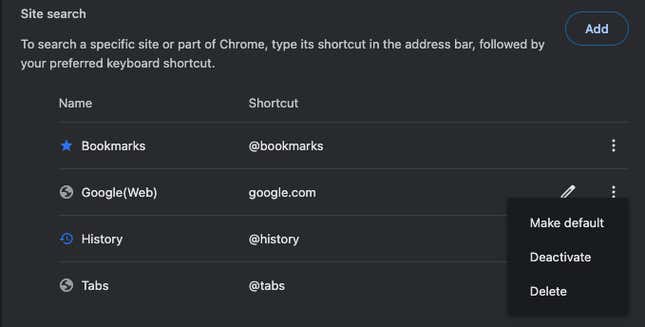
Table of Contents
This article will explore how Google uses a multi-faceted approach, leveraging diverse data sources and advanced AI techniques to maintain and improve its search AI's performance, even in the face of opt-outs. This ensures Google continues to deliver relevant search results to users worldwide.
Data Sources Beyond Indexed Web Pages
Google's AI isn't solely reliant on indexed web pages. Its training draws from a vast array of data sources, allowing for continuous improvement and adaptation regardless of individual website opt-out decisions.
Publicly Available Data
- Books: A massive corpus of text data offering diverse perspectives and knowledge.
- Academic Papers: Providing access to cutting-edge research and specialized information.
- Government Data: Offering a wealth of statistics, reports, and public records.
- Open-Source Code Repositories: A vast source of information about software and programming practices.
- Publicly accessible datasets and APIs: Providing information on a wide range of topics.
The sheer volume of this publicly available data is immense, offering a rich training ground for Google's AI. This data provides crucial context and factual information, enriching the AI's understanding of the world and enabling it to better comprehend search queries and deliver accurate results, even without access to specific opted-out websites.
User Interaction Data
Google anonymizes user interaction data to protect privacy while simultaneously using it to refine its search algorithms. This data provides invaluable feedback for improving search relevance and algorithm accuracy.
- Search Queries: The keywords users type, revealing their information needs.
- Clicks: Which search results users select, indicating relevance and user preference.
- Dwell Time: How long users spend on a given page, reflecting engagement and satisfaction.
- Mouse movements and scrolling behavior: Providing insights into user interaction with search results.
Analyzing these anonymized signals allows Google to gauge the effectiveness of its search results and iteratively improve the AI's ability to understand and respond to user intent. This is a crucial component of maintaining search quality, irrespective of website opt-out status.
Internal Google Data
Google also leverages its internal data resources to enhance its AI's capabilities.
- Google's own products and services data: Insights gleaned from Google Maps, Google Translate, and other services.
- Internal knowledge bases: Vast repositories of information accumulated over years of development.
- Proprietary datasets: Unique information sets not publicly available.
This internal data provides a crucial layer of context and understanding, refining the AI's comprehension of complex topics and relationships between different concepts. This internal knowledge base helps improve the overall accuracy and contextual understanding of the search AI.
Advanced AI Training Techniques
Google employs cutting-edge AI training techniques to maximize the utility of its diverse data sources, even when facing content opt-outs.
Unsupervised Learning
Unsupervised learning allows Google's AI to discover patterns and structures within massive datasets without explicit labels.
- Clustering: Grouping similar data points together to identify underlying themes and relationships.
- Dimensionality Reduction: Simplifying complex datasets while preserving crucial information.
These techniques are essential for analyzing the vast and varied data sources described above, allowing the AI to identify relationships and connections even within data that lacks pre-defined categories or labels. This is particularly valuable when dealing with opted-out content, where labeled data might be scarce.
Transfer Learning
Transfer learning enables Google to apply knowledge gained from one task to another.
- Knowledge gained from analyzing publicly available books can be transferred to improve the understanding of nuanced queries.
- Patterns learned from analyzing user search behavior can be applied to improve ranking algorithms.
This efficient approach allows Google to leverage the knowledge acquired from one data source to improve performance in other areas, maximizing the value of all available data, including data not directly related to specific websites.
Reinforcement Learning
Reinforcement learning allows the AI to learn through trial and error, optimizing its performance based on user feedback.
- The AI makes predictions about search relevance.
- User interactions (clicks, dwell time) provide feedback on the accuracy of those predictions.
- The AI adjusts its strategies based on this feedback to continuously improve its accuracy.
This iterative process ensures the AI constantly refines its approach, leading to increasingly relevant and accurate search results. This is an ongoing process crucial for keeping search results top-notch, regardless of website participation.
The Ethical Considerations of Opt-Out and AI Training
Google's use of data, even with content opt-outs, raises important ethical considerations.
Balancing User Privacy and AI Improvement
Google emphasizes data anonymization and robust privacy protections.
- Data is anonymized to prevent identification of individuals.
- Compliance with regulations like GDPR and CCPA is paramount.
Striking a balance between advancing AI and protecting user privacy is a critical ongoing challenge. Google actively invests in privacy-preserving technologies and adheres to strict data handling protocols.
Transparency and Accountability
Transparency regarding data usage practices is essential for building user trust.
- Google should clearly communicate how it uses data for AI training, even when content is opted out.
- Accountability mechanisms should be in place to address concerns and ensure responsible data handling.
Open communication and accountability are crucial aspects of maintaining user trust and ensuring responsible AI development.
Conclusion: Understanding Google's Search AI Training Even with Web Content Opt-Out
Google's ability to train its search AI effectively, even when websites opt out of indexing, relies on a diverse range of data sources and sophisticated AI training techniques. This includes leveraging publicly available data, user interaction data, internal Google data, and advanced methods like unsupervised learning, transfer learning, and reinforcement learning. This ensures the continuous improvement and relevance of Google's search results. However, ethical considerations regarding user privacy and transparency remain paramount, demanding ongoing attention and responsible practices from Google. To further explore this topic, delve into Google's official resources on data privacy and AI development, and continue your research on Google search AI training, Google AI and data privacy, and optimizing Google search results.
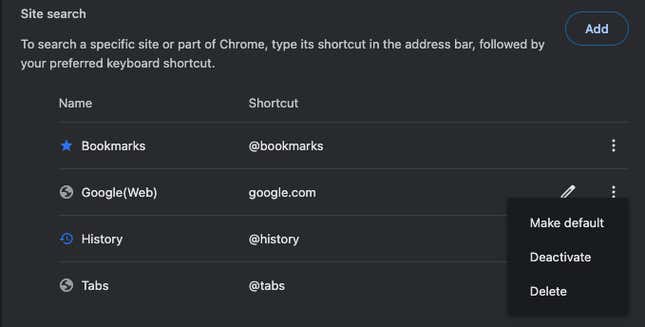
Featured Posts
-
Apo Main Event Nelson Dong Secures A 390 000 Prize
May 05, 2025 -
Honjo Sheung Wan A Modern Japanese Restaurant Review In Hong Kong
May 05, 2025 -
Murder Investigation Mother Charged With Criminal Neglect Of 16 Year Old
May 05, 2025 -
Marvels Quality Control Addressing Concerns In Film And Television
May 05, 2025 -
Partial Eclipse In Nyc This Saturday Time Viewing And Safety
May 05, 2025
Latest Posts
-
Rumours 48th Anniversary The Impact Of Personal Conflict On Fleetwood Macs Iconic Album
May 05, 2025 -
The Making Of Rumours How Fleetwood Macs Personal Lives Fueled A Legendary Album 48 Years Later
May 05, 2025 -
Novo Izdanje Gibonija Promoviranje Na Sarajevo Book Fairu
May 05, 2025 -
48 Years Of Rumours Fleetwood Macs Implosion And The Album That Defined A Generation
May 05, 2025 -
Gibonnijeva Posjeta Sarajevo Book Fairu Sto Ocekivati
May 05, 2025