Outdated Business Applications: A Roadblock To AI Success
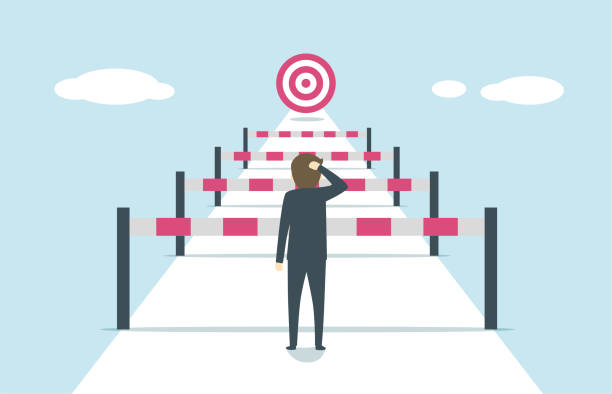
Table of Contents
Data Incompatibility and Integration Challenges
Integrating data from outdated business applications with modern AI platforms is a significant hurdle. The inherent limitations of legacy systems create significant data compatibility issues, hindering the efficient flow of information crucial for effective AI training and deployment.
- Data silos and lack of data interoperability: Many legacy systems operate in isolation, creating data silos that prevent a holistic view of business operations. This fragmented data makes it incredibly difficult to train AI models effectively.
- Inconsistent data formats and structures: Outdated systems often use different data formats and structures, making it challenging to consolidate and analyze data for AI purposes. This requires extensive data cleansing and transformation.
- Difficulty in accessing and cleaning data for AI training: Extracting, cleaning, and preparing data from legacy systems is often a time-consuming and expensive process. The data may be incomplete, inaccurate, or inconsistent, requiring significant manual intervention.
- Examples: Mainframe systems, outdated Customer Relationship Management (CRM) systems, and legacy Enterprise Resource Planning (ERP) solutions often present significant compatibility problems with modern AI tools.
The cost and time involved in data migration and integration can be substantial. However, solutions exist. Implementing data integration platforms and employing Extract, Transform, Load (ETL) processes can significantly streamline this process, paving the way for successful AI integration.
Lack of Scalability and Agility in Outdated Systems
The rigid nature of outdated business applications significantly hinders the flexibility required for effective AI model deployment and scaling. AI initiatives demand scalable and agile infrastructure, something legacy systems often lack.
- Limited processing power for AI workloads: Legacy systems often struggle to handle the computational demands of AI algorithms, leading to slow processing times and inefficient model training.
- Inability to handle large datasets efficiently: AI models require large datasets for training. Outdated systems often lack the capacity to store, process, and manage these datasets effectively.
- Difficulty in adapting to evolving AI technologies: The rapid pace of innovation in AI means that new technologies and algorithms emerge constantly. Legacy systems are often difficult to adapt to these advancements.
- Challenges in deploying AI models to different environments: Deploying AI models across multiple environments (on-premises, cloud, edge) can be challenging with inflexible legacy systems.
The need for scalable cloud infrastructure is paramount for AI success. Cloud-native applications, designed for scalability and agility, offer a superior alternative to legacy systems, enabling businesses to easily deploy and scale AI models as needed.
Security Risks Posed by Outdated Business Applications
Outdated business applications represent significant security vulnerabilities, increasing the risk of cyber threats and data breaches. This jeopardizes not only business operations but also the integrity of AI initiatives.
- Lack of up-to-date security patches and features: Legacy systems often lack the latest security patches and features, making them vulnerable to known exploits.
- Increased risk of malware infections: Outdated software is a prime target for malware infections, potentially leading to data loss and system disruption.
- Potential for data leakage and non-compliance with data privacy regulations (GDPR, CCPA): Insecure legacy systems can expose sensitive data to unauthorized access, resulting in significant legal and financial consequences.
- Difficulty in integrating robust security measures with outdated systems: Integrating modern security tools and technologies with legacy systems is often difficult and may compromise system stability.
Insecure systems directly compromise AI implementation due to trust and data integrity issues. Robust security protocols and regular security audits are essential for mitigating these risks and ensuring the successful integration of AI.
The High Cost of Maintaining Outdated Systems
Maintaining and supporting outdated business applications incurs significant financial burdens. These costs often outweigh the benefits of clinging to legacy systems, especially when considering the potential ROI of modernization.
- High maintenance costs due to lack of vendor support: Many legacy systems are no longer supported by vendors, leading to increased maintenance costs and difficulty in finding skilled personnel to maintain them.
- Increased IT staff time spent on troubleshooting and patching: IT staff spend significant time troubleshooting issues and applying patches to outdated systems, diverting resources from more strategic initiatives.
- Opportunity cost of not investing in modern systems capable of supporting AI: The opportunity cost of not investing in modern systems that can support AI is substantial. Businesses risk falling behind competitors who have embraced AI-driven solutions.
The Total Cost of Ownership (TCO) of legacy systems often far exceeds that of modern solutions. Upgrading to AI-ready systems provides a significant Return on Investment (ROI) by improving efficiency, reducing operational costs, and unlocking new revenue streams through AI-powered innovation.
Conclusion: Overcoming the Roadblock to AI Success
Outdated business applications present significant challenges to successful AI adoption, including data incompatibility, scalability limitations, security risks, and high maintenance costs. Modernizing legacy systems is crucial for unlocking the true potential of AI.
Businesses need to assess their existing IT infrastructure, identify legacy systems hindering AI integration, and develop a comprehensive modernization plan. This may involve migrating to cloud-based solutions, implementing data integration platforms, and investing in robust security measures. By proactively addressing these challenges, businesses can pave the way for successful AI implementation and gain a competitive edge in the rapidly evolving digital landscape.
Ready to evaluate your own outdated business applications and unlock the transformative power of AI? [Link to relevant resource, e.g., a case study or consultation service]
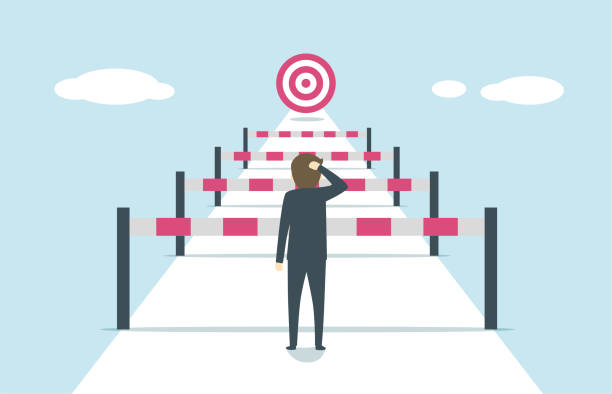
Featured Posts
-
Olivia Wilde And Dane Di Liegro Spotted Again Looking Loved Up At Lakers Game
May 01, 2025 -
Best In Klas Nrc Health Leads In Healthcare Experience Management
May 01, 2025 -
Tahun Ini Bkpm Bidik Investasi Rp3 6 Triliun Di Pekanbaru
May 01, 2025 -
Incredibile Rimonta Della Flaminia Ora E Seconda
May 01, 2025 -
Ripple Sec Lawsuit Potential Commodity Classification For Xrp In Settlement
May 01, 2025
Latest Posts
-
Un Accompagnement Numerique Pour Vos Thes Dansants
May 02, 2025 -
Australias Rugby Struggle A Former Wallabys Perspective Phipps
May 02, 2025 -
England Vs France Six Nations Dalys Late Score Seals Victory
May 02, 2025 -
France Dominates Italy In Six Nations Eyes Ireland Next
May 02, 2025 -
Nikki Burdine And Neil Orne Two New Projects Together
May 02, 2025