Quantum Computing (QBTS) And AI: A New Era For Pharmaceutical Innovation
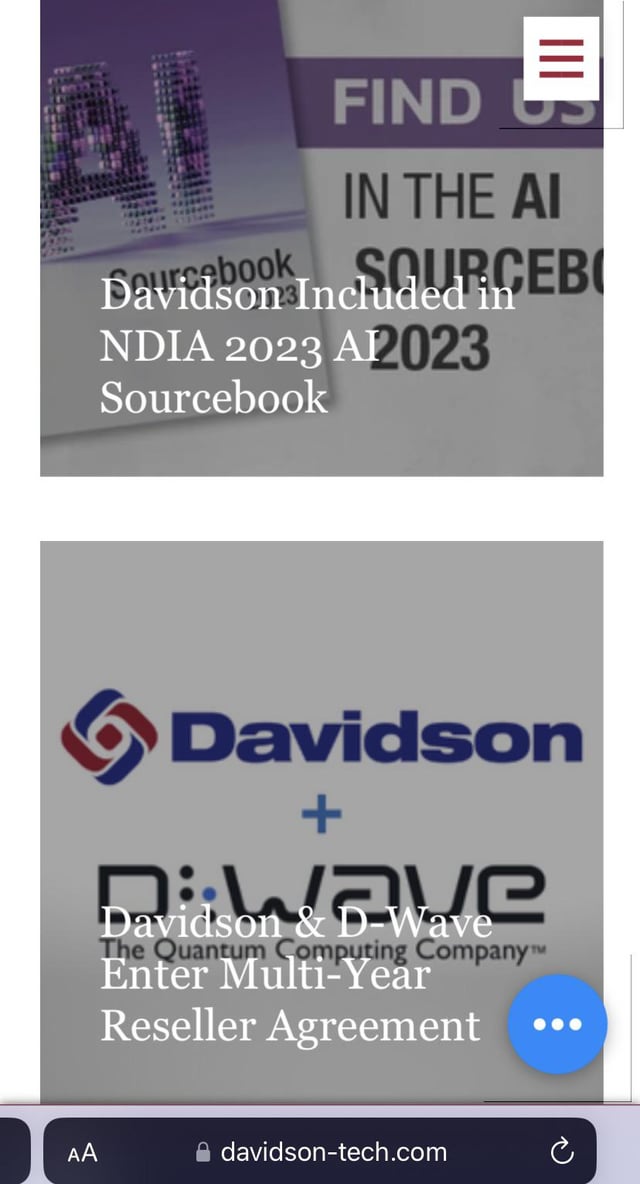
Table of Contents
Accelerating Drug Discovery with Quantum Computing and AI
The integration of QBTS and AI is poised to significantly accelerate the drug discovery process, traditionally a lengthy and costly endeavor. This acceleration is achieved through advancements in two key areas: enhanced molecular modeling and simulation, and optimized drug design and synthesis.
Enhanced Molecular Modeling and Simulation
Quantum computers, with their ability to handle exponentially larger datasets than classical computers, are revolutionizing molecular modeling. QBTS algorithms can simulate molecular interactions with unprecedented accuracy, providing a far more detailed understanding of how drug molecules interact with their targets. This improved accuracy leads to better predictions of drug efficacy and side effects, minimizing the risk of failure in later stages of development. AI, meanwhile, plays a crucial role in analyzing the vast datasets generated by these simulations. Machine learning algorithms can identify patterns and relationships that would be impossible for humans to detect, leading to the identification of promising drug candidates and the optimization of their design.
- Improved prediction of protein folding: Understanding protein folding is critical for drug discovery, and QBTS offers a powerful tool for simulating this complex process with greater accuracy.
- Faster virtual screening of compounds: QBTS can significantly speed up the virtual screening of large compound libraries, identifying potential drug candidates much faster than traditional methods.
- Accurate estimation of drug-target binding affinities: Precise prediction of binding affinities is crucial for determining a drug's potency. QBTS offers the potential for significantly improved accuracy in these estimations.
Optimizing Drug Design and Synthesis
AI-powered algorithms, trained on the data generated by QBTS simulations, can design novel drug molecules with improved properties, such as increased efficacy and reduced toxicity. This iterative process, combining the power of quantum simulation with the learning capabilities of AI, enables the development of drugs tailored to specific targets and patient populations. Furthermore, quantum computing can optimize the synthesis pathways for complex drug molecules, leading to more efficient and cost-effective production.
- Development of personalized medicines: By modeling individual patient responses, QBTS and AI can contribute to the development of truly personalized therapies.
- Efficient synthesis of complex molecules: Quantum algorithms can help optimize chemical reactions, leading to faster and more efficient synthesis of complex drug molecules.
- Reduced trial-and-error in drug development: By accurately predicting the properties of drug candidates, QBTS and AI can significantly reduce the need for costly and time-consuming trial-and-error experimentation.
Revolutionizing Clinical Trials with AI and QBTS
The impact of QBTS and AI extends beyond drug discovery to clinical trials, promising to improve efficiency and reduce costs. This transformation is primarily driven by improved patient stratification and the ability to predict clinical trial outcomes.
Patient Stratification and Personalized Medicine
AI algorithms can analyze vast amounts of patient data, including genetic information, medical history, and lifestyle factors, to identify subgroups who are most likely to benefit from a particular drug. This allows for the recruitment of more suitable participants in clinical trials, increasing the likelihood of success and reducing the risk of adverse events. QBTS can further enhance this process by modeling individual patient responses to drugs, enabling the development of personalized therapies tailored to specific patient characteristics.
- Improved patient recruitment for clinical trials: By accurately identifying suitable patients, AI can significantly improve the efficiency of patient recruitment for clinical trials.
- Reduced costs and time associated with trials: More efficient patient selection leads to fewer trial failures and a faster path to market for successful drugs.
- Increased efficacy of treatments: Personalized medicine, facilitated by QBTS and AI, leads to more effective treatments with fewer side effects.
Predicting Clinical Trial Outcomes
The combination of AI and QBTS can analyze massive datasets from previous clinical trials to identify patterns and predictors of success or failure. This predictive capability allows researchers to make more informed decisions about which drugs to advance into clinical trials, reducing the risk of investing resources in projects unlikely to succeed.
- More efficient allocation of resources: Predictive models can help prioritize the most promising drug candidates, optimizing resource allocation.
- Improved decision-making in drug development: Data-driven insights improve the overall decision-making process throughout drug development.
- Reduced overall costs: By avoiding costly failures, the integration of AI and QBTS can significantly reduce the overall cost of drug development.
Addressing the Challenges of Quantum Computing in Pharmaceutical Applications
Despite the immense potential, the application of QBTS in pharmaceutical research faces several challenges that need to be addressed.
Hardware Limitations and Scalability
Current QBTS technology is still in its early stages of development. The available quantum computers have limited qubit numbers and coherence times, restricting their scalability and applicability to complex pharmaceutical problems. Significant advancements in hardware are needed to overcome these limitations.
- Need for increased qubit coherence: Longer qubit coherence times are crucial for performing more complex calculations.
- Development of fault-tolerant quantum computers: Fault tolerance is essential for reliable computation on larger-scale quantum computers.
- Improved error correction techniques: Effective error correction methods are needed to mitigate the effects of noise in quantum computations.
Data Management and Algorithm Development
Handling and processing the vast amounts of data generated by QBTS and AI requires sophisticated data management strategies and robust computational infrastructure. Furthermore, the development of efficient quantum algorithms tailored to specific pharmaceutical applications is crucial for realizing the full potential of this technology.
- Need for advanced data infrastructure: High-performance computing clusters and specialized data storage solutions are needed to handle large datasets.
- Development of specialized quantum algorithms: Efficient quantum algorithms need to be developed for specific tasks in drug discovery and development.
- Collaboration between researchers: Collaboration between experts in quantum computing, AI, and pharmaceutical sciences is essential for successful implementation.
Conclusion
The integration of quantum computing (QBTS) and AI presents an unprecedented opportunity to revolutionize pharmaceutical innovation. By accelerating drug discovery, optimizing drug design, and enhancing clinical trials, this powerful combination promises to deliver safer, more effective, and more personalized medicines to patients faster than ever before. While challenges remain, the potential benefits of this transformative technology are undeniable. To fully realize this potential, continued investment in QBTS research, algorithm development, and data infrastructure is vital. Embracing the possibilities of quantum computing (QBTS) and AI will usher in a new era of breakthroughs in the pharmaceutical industry. Let's work together to accelerate this exciting field and unlock the true power of quantum computing (QBTS) in pharmaceutical innovation.
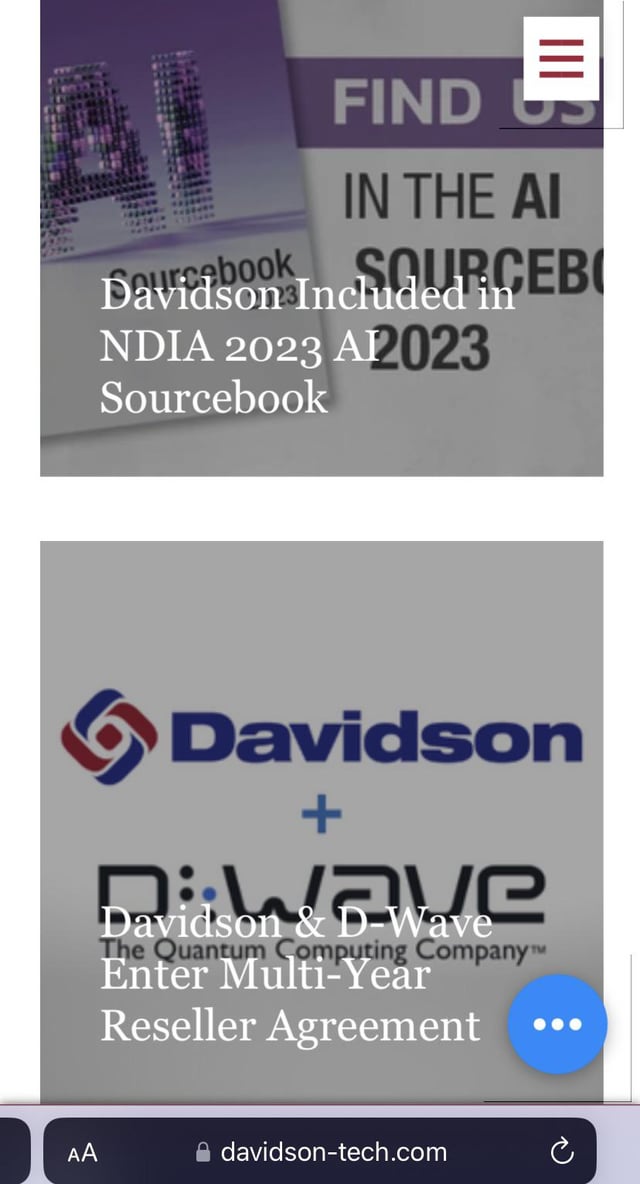
Featured Posts
-
Man Utd Transfer News Red Devils Target Premier League Forward Newcastle Competition
May 20, 2025 -
Biarritz Trois Journees D Echanges Sur Le Role Des Femmes
May 20, 2025 -
Nyt Mini Crossword Answers April 2 Complete Solutions
May 20, 2025 -
Arsenal Transfer News Gunners To Battle Liverpool For Premier League Star
May 20, 2025 -
Germanys 5 4 Aggregate Victory Over Italy Sends Them To Nations League Final Four
May 20, 2025
Latest Posts
-
Britains Got Talent David Walliams And Simon Cowells Public Feud Explodes
May 20, 2025 -
David Walliams What Happened On Britains Got Talent
May 20, 2025 -
Walliams Slams Cowell Amidst Britains Got Talent Dispute
May 20, 2025 -
David Walliams Scathing Simon Cowell Takedown A Britains Got Talent Feud
May 20, 2025 -
David Walliams Fing Production Greenlit By Stan
May 20, 2025