Revealing The Mechanisms Behind AI's Apparent Thought
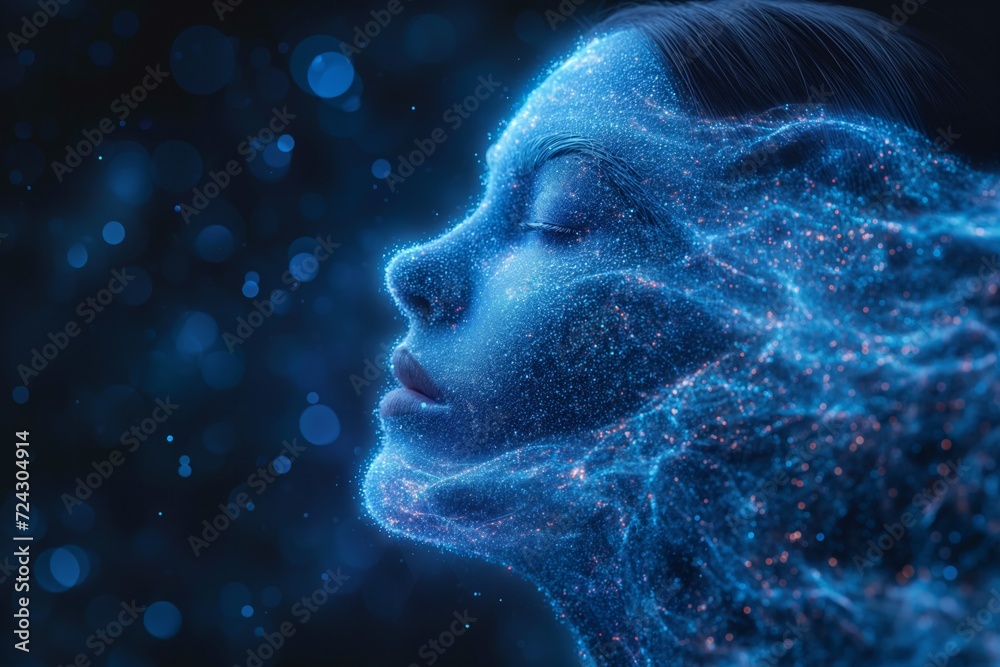
Table of Contents
The Illusion of Understanding: How AI Processes Information
AI's seemingly intelligent actions often stem from its ability to identify patterns within massive datasets. This is particularly true for machine learning algorithms, which form the backbone of many modern AI applications. Let's examine how this process works and its inherent limitations.
Statistical Pattern Recognition
At its core, much of AI relies on sophisticated statistical pattern recognition. Algorithms, like neural networks and decision trees, analyze vast amounts of data to identify correlations and make predictions.
- Neural Networks: These systems, inspired by the structure of the human brain, consist of interconnected nodes processing information in layers. They excel at tasks like image recognition, identifying patterns in pixel data to classify objects.
- Decision Trees: These algorithms create a branching structure based on data features, leading to a final classification or prediction. They are often used in simpler AI applications where interpretability is crucial.
However, this pattern recognition doesn't necessarily equate to true understanding. AI systems might correctly identify a cat in an image, but they lack the genuine comprehension of what a cat is—its biology, behavior, and place in the world.
- Lack of Contextual Understanding: AI often struggles with situations requiring broader context or common sense reasoning. A system might correctly translate a sentence but fail to grasp the underlying nuances of meaning.
- Limited Generalization: An AI trained to identify cats in one specific setting may struggle to recognize them in different environments or under varying lighting conditions.
The Role of Big Data
The impressive performance of modern AI is inextricably linked to the availability of massive datasets. These datasets fuel the learning process, allowing algorithms to identify subtle patterns and refine their predictions.
- Data Bias: A significant challenge lies in the potential for bias within these datasets. If the training data reflects existing societal biases, the AI system will likely perpetuate and even amplify these biases in its outputs. This is a critical concern in areas like facial recognition and loan applications.
- Data Volume and Accuracy: Generally, larger datasets lead to more accurate and robust AI models. However, simply increasing data volume doesn't guarantee improved performance. The quality and diversity of the data are equally crucial.
Decoding the "Black Box": Understanding AI Decision-Making Processes
Many advanced AI models, especially deep neural networks, function as "black boxes." Their internal workings are often opaque, making it difficult to understand how they arrive at their conclusions. This lack of transparency poses challenges for trust, accountability, and responsible deployment.
Explainability and Interpretability in AI
The field of "Explainable AI" (XAI) is actively working to address this issue. The goal is to develop techniques to make AI decision-making processes more transparent and understandable.
- Explainable AI (XAI) Techniques: Researchers are exploring various methods to shed light on the inner workings of AI models.
- Model Interpretation Techniques: Methods like LIME (Local Interpretable Model-agnostic Explanations) and SHAP (SHapley Additive exPlanations) aim to provide insights into individual predictions, highlighting the features that most influenced the AI's decision.
The Limits of Symbolic Reasoning
Human intelligence relies heavily on symbolic reasoning and logical deduction. We use symbols to represent concepts, allowing us to manipulate and reason about information in abstract ways. AI, on the other hand, predominantly uses statistical methods.
- Common Sense Reasoning: AI systems often struggle with tasks requiring common sense reasoning. A human child understands intuitively that an object cannot pass through a solid wall, but AI might require explicit programming to account for such limitations.
- Contextual Understanding and Abstract Thought: AI often fails in situations that require a nuanced understanding of context and abstract thought. Understanding metaphors, sarcasm, or complex social interactions remains a challenge.
The Future of AI and the Nature of Thought
Despite the current limitations, ongoing research is pushing the boundaries of AI capabilities. The pursuit of more human-like AI is driving innovation in several key areas.
Advancements in AI Research
Researchers are exploring new techniques to enhance AI's capabilities, moving beyond simple pattern recognition.
- Reinforcement Learning: This approach trains AI agents to learn through trial and error, interacting with an environment and receiving rewards or penalties based on their actions.
- Transfer Learning: This allows AI models trained for one task to adapt more easily to new tasks, reducing the need for extensive retraining.
- Neuro-Symbolic AI: This combines the strengths of neural networks (pattern recognition) and symbolic AI (logical reasoning), aiming to create more robust and adaptable systems.
These advancements hold the potential to create AI systems exhibiting more sophisticated forms of "thought," but significant challenges remain.
Ethical Considerations
The development of increasingly intelligent AI systems raises profound ethical questions.
- Bias and Fairness: Addressing bias in AI is crucial to ensure equitable outcomes and avoid perpetuating harmful societal inequalities.
- Accountability and Transparency: Determining responsibility for AI's actions, particularly in high-stakes applications, requires careful consideration.
- Potential for Misuse: The power of advanced AI systems could be exploited for malicious purposes, emphasizing the need for careful regulation and ethical guidelines. The potential for artificial consciousness and sentience also necessitates careful philosophical and ethical debate.
Conclusion
AI's apparent thought is a powerful illusion, built on sophisticated statistical pattern recognition and fueled by massive datasets. While current AI systems demonstrate remarkable capabilities, they lack the genuine understanding, common sense reasoning, and abstract thought characteristic of human intelligence. Understanding the mechanisms behind AI's apparent thought is crucial for responsible development and deployment. Continue your exploration of this fascinating field by exploring the resources available at [link to relevant resources]. The future of AI, and its impact on society, hinges on our ability to develop ethical, transparent, and truly intelligent systems, going far beyond simply mimicking the appearance of thought.
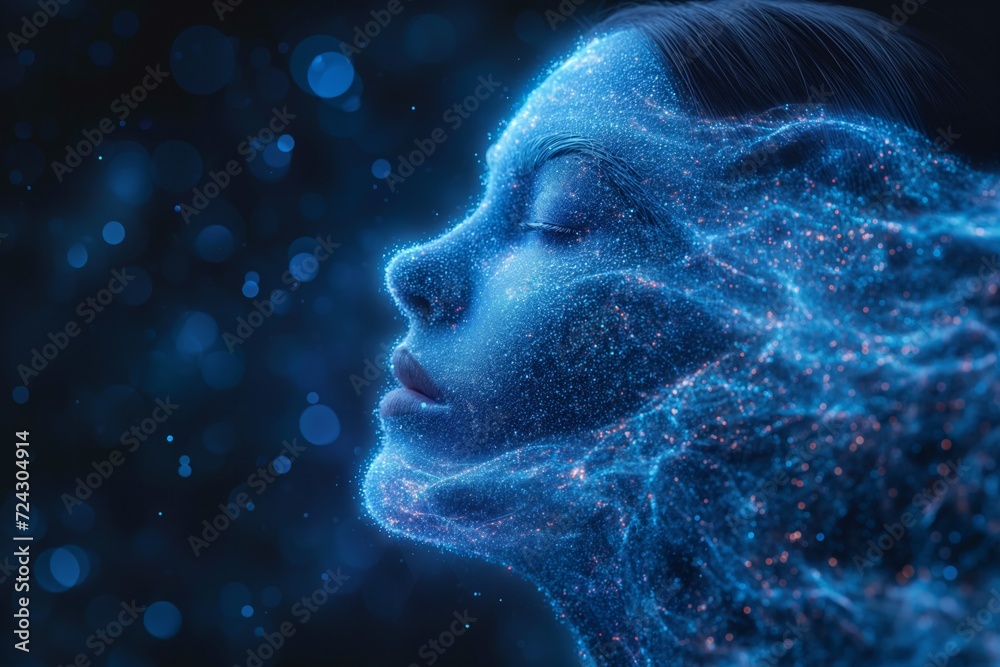
Featured Posts
-
Willie Nelsons Health A Look At The Demands Of His Touring Lifestyle
Apr 29, 2025 -
Goldman Sachs Exclusive Tariff Advice Navigating Trumps Trade Policies
Apr 29, 2025 -
Did Trumps China Tariffs Hurt The Us Economy A Look At Inflation And Supply Chains
Apr 29, 2025 -
Rare Porsche 911 S T With Pts Riviera Blue Paint For Sale
Apr 29, 2025 -
Securing Your Capital Summertime Ball 2025 Tickets A Practical Guide
Apr 29, 2025