The Surprising Simplicity Of AI's "Thinking"
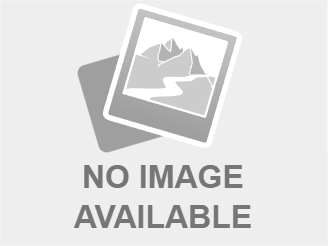
Table of Contents
The Foundation: Algorithms and Data
AI's "thinking," at its core, is based on the interplay of algorithms and data. Understanding this fundamental relationship is key to understanding how AI works.
Algorithms as Recipes
Think of AI algorithms as sophisticated recipes. These recipes, written in code, provide a precise set of instructions for processing data. They take input data, follow a series of steps, and produce an output. The "ingredients" are the data, and the "chef" is the algorithm.
- Examples of simple algorithms: Sorting a list of numbers from smallest to largest, or searching for a specific item in a database, are examples of simple algorithms. More complex AI algorithms, however, can involve millions or even billions of instructions.
- The role of different algorithm types: Supervised learning algorithms learn from labeled data (e.g., images labeled as "cat" or "dog"), while unsupervised learning algorithms find patterns in unlabeled data. Reinforcement learning algorithms learn through trial and error, receiving rewards or penalties for their actions.
- How algorithms learn from data: Many AI algorithms learn by adjusting their internal parameters based on the data they process. This process, often called "training," allows the algorithm to improve its performance over time.
The Importance of Data
High-quality data is the lifeblood of AI. Without sufficient, relevant, and accurate data, even the most sophisticated algorithm will fail. The process of preparing data for AI is crucial and involves several steps.
- Types of data used in AI: AI systems use various types of data, including structured data (organized in tables) and unstructured data (text, images, audio, video).
- The impact of biased or incomplete data: Biased or incomplete data can lead to AI systems that make unfair or inaccurate predictions. For example, an AI trained on biased data might perpetuate existing societal biases.
- The need for large datasets: Many advanced AI techniques, particularly deep learning, require massive datasets to achieve good performance. The more data, the better the AI can learn and make accurate predictions. This is why data acquisition and cleaning are crucial steps in the development of most AI systems.
Pattern Recognition: The Core of AI's "Thinking"
AI's ability to identify patterns is the cornerstone of its seemingly intelligent behavior. This process involves sophisticated statistical analysis and machine learning techniques.
Identifying Patterns
AI systems identify patterns in data by searching for statistical regularities and correlations. For example, an image recognition system might identify patterns in pixel data to recognize a face, while a natural language processing system might identify patterns in word sequences to understand the meaning of a sentence.
- Examples of pattern recognition: Image recognition (identifying objects in images), natural language processing (understanding and generating human language), fraud detection (identifying unusual financial transactions), medical diagnosis (identifying patterns in medical images or patient data).
- The concept of statistical significance: AI algorithms assess the statistical significance of identified patterns, determining whether the patterns are likely to be real or due to random chance.
From Patterns to Predictions
Once AI systems identify patterns, they use these patterns to make predictions and decisions. This involves inferring relationships between different data points and extrapolating these relationships to new, unseen data.
- How AI makes inferences from patterns: AI systems use statistical models and machine learning algorithms to make inferences from the identified patterns. This allows them to predict future events or make decisions based on the data they have processed.
- The role of probability in AI predictions: AI predictions are often expressed as probabilities, reflecting the uncertainty inherent in making predictions based on limited data.
- Limitations of AI predictions: AI predictions are only as good as the data and algorithms they are based on. They can be inaccurate or biased if the data is flawed or the algorithm is poorly designed.
Debunking the Myth of Consciousness
While AI can perform impressive feats, it's crucial to understand that it doesn't "think" in the same way humans do. AI lacks the subjective experience, emotions, and self-awareness that characterize human consciousness.
AI vs. Human Intelligence
The key difference lies in the nature of intelligence. Human intelligence is characterized by consciousness, self-awareness, emotions, and subjective experience. AI, on the other hand, is a sophisticated system of pattern recognition and prediction.
- AI lacks subjective experiences, emotions, and self-awareness: AI systems operate solely based on algorithms and data; they don't have feelings, beliefs, or desires.
- The difference between reactive and proactive AI: Reactive AI systems respond directly to their environment, while proactive AI systems can plan and make decisions based on anticipated future events. Even the most advanced proactive AI systems, however, lack the rich inner life of a human being.
- The ongoing debate about AI sentience: The question of whether AI can ever achieve genuine consciousness is a topic of ongoing debate among scientists and philosophers.
The Illusion of Complexity
The complexity of AI applications often stems from the scale and sophistication of the algorithms and data used, not from inherent complexity within the AI itself. Large language models, for instance, are capable of generating human-quality text but rely on relatively simple statistical processes at their core.
- The role of large language models: Large language models process vast amounts of text data to learn patterns in language and generate coherent text.
- The impact of neural networks: Neural networks, a type of algorithm inspired by the human brain, can learn complex patterns but are still fundamentally based on simple mathematical operations.
- How seemingly complex outputs arise from relatively simple processes: The seemingly complex outputs of AI systems are often the result of combining many simple processes at a massive scale.
Conclusion
In summary, AI's "thinking" is based on algorithms processing data to identify patterns and make predictions. It's not conscious thought, but a sophisticated form of pattern recognition. While AI applications can be incredibly complex, the underlying processes are surprisingly simple. Understanding this distinction is crucial for responsibly developing and deploying AI systems. The key takeaway is that while the outputs of AI can seem remarkably complex and even human-like, the processes underlying these outputs are based on relatively straightforward mathematical and statistical principles.
Understanding the surprising simplicity of AI's thinking is crucial for navigating the future of technology. Learn more about the fascinating world of AI algorithms and their capabilities today! Explore the nuances of AI's thought processes and the ethical considerations surrounding their development and deployment.
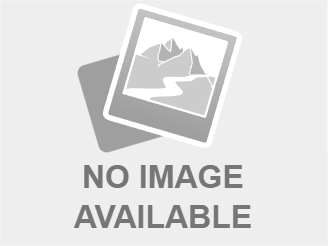
Featured Posts
-
Attorney Generals Transgender Sports Ban Minnesotas Response Crucial
Apr 29, 2025 -
Trumps Trade War With China Winners And Losers In The Us Economy
Apr 29, 2025 -
Hagia Sophia History Structure And Survival Of An Icon
Apr 29, 2025 -
Trump Funding Cuts Spark Global Scramble For Us Scientific Expertise
Apr 29, 2025 -
Office365 Executive Inboxes Targeted Millions Stolen Fbi Alleges
Apr 29, 2025
Latest Posts
-
New Music Willie Nelson Releases 77th Solo Album At 91
Apr 29, 2025 -
Willie Nelson Drops New Album Before Turning 92
Apr 29, 2025 -
New Willie Nelson Album A 77th Solo Effort Before His 92nd Birthday Celebration
Apr 29, 2025 -
New Documentary Showcases Willie Nelsons Respect For His Roadies
Apr 29, 2025 -
Willie Nelson Releases 77th Solo Album Ahead Of 92nd Birthday
Apr 29, 2025