AI And Quantum Computing In Drug Discovery: D-Wave (QBTS) Leading The Charge
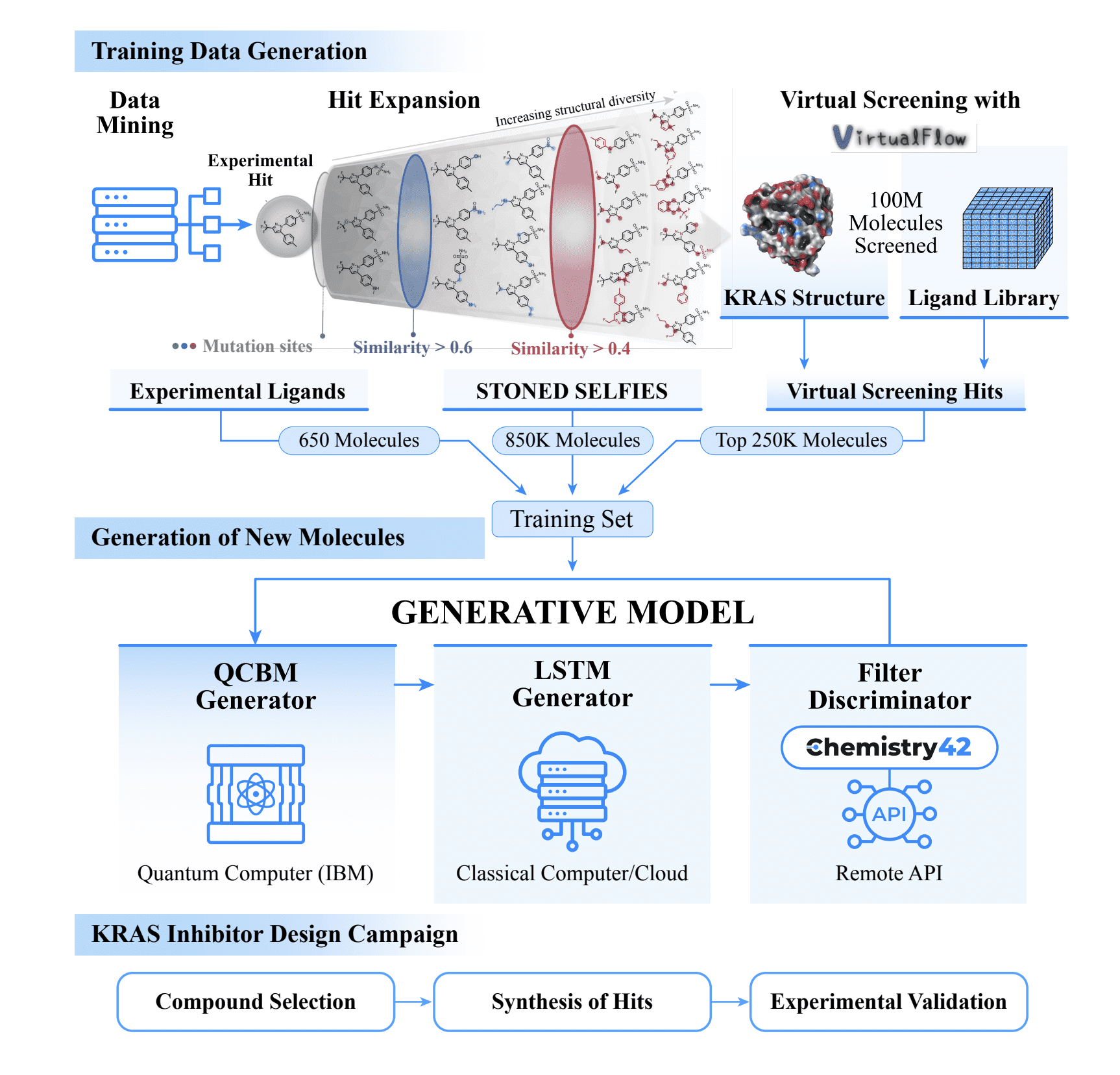
Table of Contents
AI's Role in Drug Discovery
Artificial intelligence is rapidly transforming drug discovery, offering powerful tools at each stage of the development pipeline.
Target Identification and Validation
AI algorithms excel at analyzing massive datasets – genomics, proteomics, clinical trial data – to identify promising drug targets. These targets, often specific proteins or genes, are crucial for treating diseases.
- AI Techniques: Machine learning (ML), deep learning (DL), and other advanced algorithms are used to identify patterns and relationships within complex biological data.
- Advantages: AI significantly increases speed and accuracy while reducing the cost associated with traditional target identification methods.
- Examples: AI has successfully identified novel targets for cancer, Alzheimer's disease, and other challenging conditions, accelerating the development of targeted therapies.
Lead Compound Optimization
Once potential drug targets are identified, AI plays a crucial role in designing and optimizing lead compounds – the initial drug candidates.
- AI Techniques: Generative models can design novel molecules with desired properties, while reinforcement learning algorithms optimize these molecules for efficacy and minimize potential side effects.
- Tools: Numerous AI-powered platforms and software packages are now available to assist in lead optimization, automating many tedious and time-consuming tasks.
- Examples: AI has been instrumental in improving the potency and selectivity of numerous drug candidates, resulting in more effective and safer medications.
Predicting Drug Interactions and Toxicity
Predicting potential drug interactions and toxicity is vital for ensuring patient safety and reducing the risk of adverse events. AI algorithms significantly enhance this process.
- AI Techniques: AI models can predict drug interactions and toxicity based on various factors, including molecular structure, pharmacokinetic properties, and genetic information. This reduces reliance on extensive and costly animal testing.
- Impact on Preclinical Testing: AI-driven toxicity prediction allows for a more efficient allocation of resources, focusing on the most promising candidates and reducing the need for extensive animal testing.
- Improved Success Rates: Data suggests that AI-driven toxicity prediction has significantly improved the success rate of drug candidates progressing through clinical trials.
Quantum Computing's Potential in Drug Discovery
Quantum computing, with its ability to handle exponentially complex calculations, holds immense promise for drug discovery.
Solving Complex Molecular Simulations
Simulating the behavior of molecules is computationally demanding. Quantum computers offer a significant advantage over classical computers by handling these complex calculations with substantially greater speed and accuracy.
- Advantages: Quantum computing can simulate molecular interactions with unprecedented precision, leading to a better understanding of drug mechanisms and improved drug design.
- Quantum Algorithms: Quantum annealing and variational quantum eigensolver (VQE) are examples of quantum algorithms applicable to drug discovery.
- Handling Large Datasets: Quantum computers are particularly well-suited for analyzing the enormous datasets generated in drug discovery research.
Accelerating Drug Design and Optimization
Quantum computing can significantly accelerate the process of designing and optimizing drug molecules.
- Quantum Algorithms for Drug Design: Several quantum algorithms are under development to enhance the efficiency and accuracy of drug design, such as quantum machine learning algorithms.
- Discovering Novel Drug Candidates: The power of quantum computing opens possibilities to discover novel drug candidates that are inaccessible using classical methods.
- Faster Development Times: By drastically reducing computation time for complex molecular simulations, quantum computing has the potential to significantly shorten drug development timelines.
Enhancing AI Algorithms
Quantum computing can further enhance the performance of AI algorithms used in drug discovery.
- Hybrid Classical-Quantum Approaches: Integrating quantum computing with classical AI methods creates hybrid approaches that leverage the strengths of both technologies.
- Improved Accuracy and Efficiency: These hybrid approaches promise to improve the accuracy and efficiency of AI-based drug discovery tools.
- Quantum Machine Learning: This emerging field aims to develop quantum algorithms that can outperform classical machine learning algorithms in certain drug discovery tasks.
D-Wave's (QBTS) Contributions
D-Wave Systems, with its unique quantum annealing technology, is making significant contributions to the field of AI and quantum computing in drug discovery.
Quantum Annealing Technology
D-Wave's quantum annealers are specialized quantum computers designed to solve optimization problems efficiently. This is crucial for many aspects of drug discovery.
- Applications in Drug Discovery: D-Wave's technology can be used to optimize drug molecule designs, identify optimal drug targets, and analyze complex biological networks.
- Partnerships and Collaborations: D-Wave is actively collaborating with pharmaceutical companies and research institutions to apply its technology to real-world drug discovery challenges.
- Specific Examples: D-Wave has participated in various projects exploring the application of quantum computing to drug design and optimization, showcasing the potential of this technology.
Hybrid Classical-Quantum Approaches
D-Wave's approach focuses on integrating its quantum annealers with classical AI algorithms, leveraging the advantages of both.
- Benefits of Hybrid Approach: This hybrid approach combines the strengths of classical AI's data analysis capabilities with the power of quantum computing for solving complex optimization problems.
- Successful Applications: D-Wave has successfully demonstrated the effectiveness of this approach in solving challenging drug discovery problems.
- Scalability: The hybrid approach allows for scaling up the computational power as needed, making it suitable for increasingly complex drug discovery tasks.
Future Prospects and Potential
D-Wave's technology holds immense potential for revolutionizing the drug discovery process.
- Anticipated Improvements: Future advancements in quantum annealing technology and hybrid classical-quantum approaches promise further improvements in speed, accuracy, and efficiency.
- Potential Breakthroughs: D-Wave's continued development and collaborations have the potential to lead to significant breakthroughs in drug discovery, accelerating the development of new therapies.
- Impact on Healthcare: The impact of these advancements could be transformative, leading to faster development of new treatments for various diseases and improving the lives of patients worldwide.
Conclusion
AI and quantum computing are poised to revolutionize the drug discovery process, offering faster, cheaper, and more efficient methods for developing new medicines. D-Wave (QBTS), with its innovative quantum annealing technology and hybrid classical-quantum approaches, is playing a leading role in this exciting field. Learn more about how D-Wave (QBTS) is leading the charge in using AI and quantum computing in drug discovery and explore the future of pharmaceutical innovation. Visit [link to D-Wave's website] to discover more.
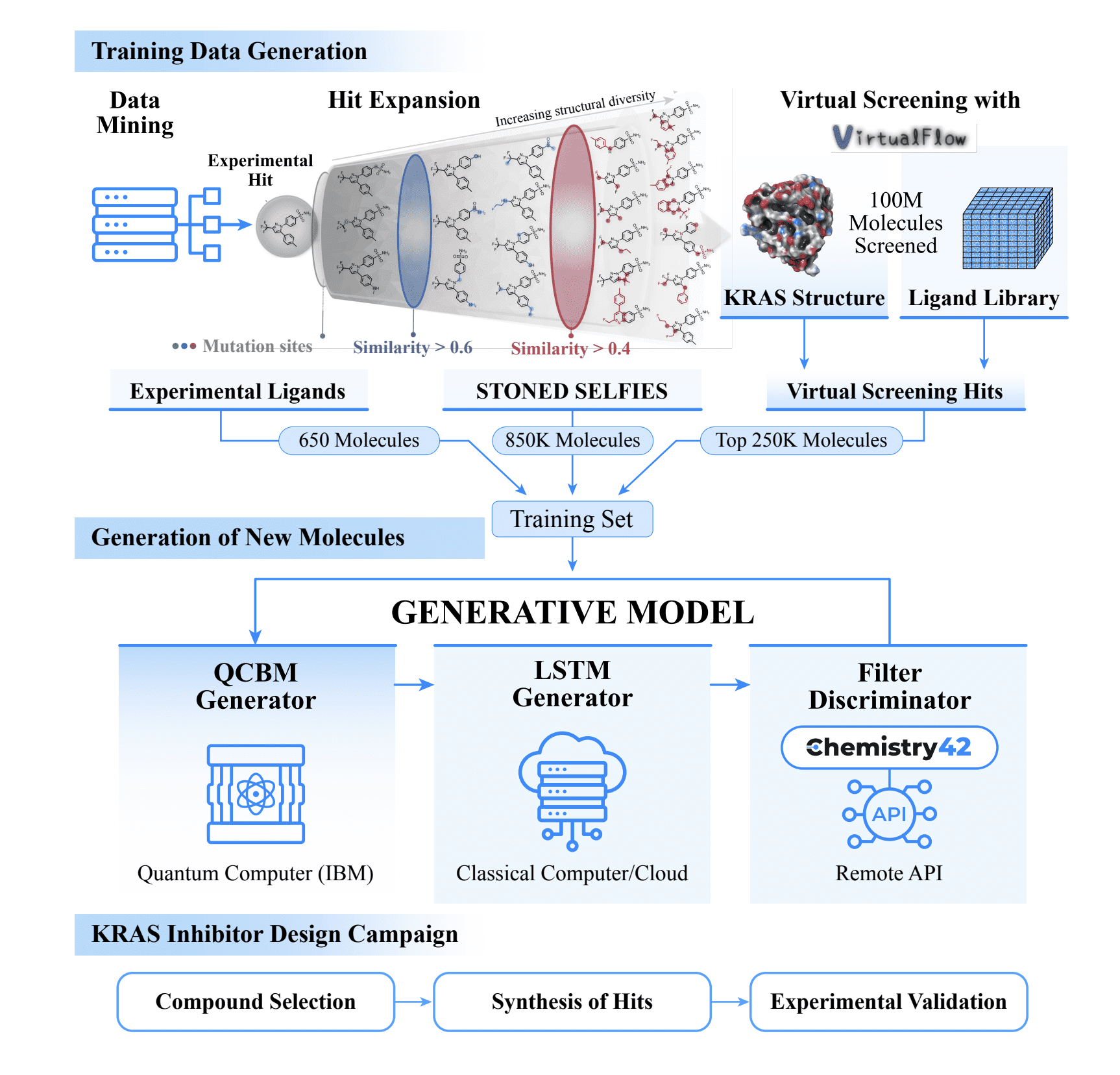
Featured Posts
-
Fremantles Q1 Revenue A 5 6 Drop Explained
May 21, 2025 -
Analyzing Qbts Stock Performance Following Earnings Releases
May 21, 2025 -
Peppa Pigs Family Grows Baby Gender Reveal
May 21, 2025 -
Dexter Resurrections Villain A Deeper Look At Their Popularity
May 21, 2025 -
Court Upholds Sentence Against Councillors Wife For Social Media Post
May 21, 2025
Latest Posts
-
Mum Jailed For Tweet After Southport Stabbing Homelessness Sentence
May 22, 2025 -
Decision Delayed Ex Tory Councillors Wifes Appeal Over Racial Hatred Tweet
May 22, 2025 -
Wife Of Jailed Tory Councillor Says Fire Rant Against Migrant Hotels Wasnt Intended To Incite Violence
May 22, 2025 -
Sentence Appeal For Ex Tory Councillors Wife Over Racist Tweet
May 22, 2025 -
Update Ex Tory Councillors Wifes Appeal On Racial Hatred Tweet
May 22, 2025