Creating Ethical And Inclusive AI: A Conversation With Microsoft's Design Chief
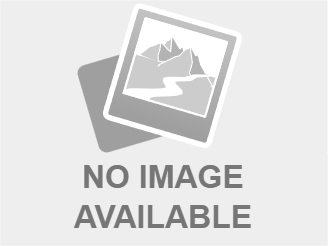
Table of Contents
Addressing Bias in AI Algorithms
AI systems, powerful as they are, are only as good as the data they are trained on. This means that biased data inevitably leads to biased outcomes, perpetuating and even amplifying existing societal inequalities. Understanding and addressing this bias is paramount for creating ethical AI.
Identifying Sources of Bias
AI bias stems from various sources within the data itself. These include:
- Sampling bias: Datasets often fail to accurately represent the full diversity of the population they aim to serve. For example, a facial recognition system trained primarily on images of light-skinned individuals will likely perform poorly on darker-skinned individuals.
- Measurement bias: Inconsistent or flawed data collection methods can introduce significant bias. Consider surveys with leading questions or medical data collected with inconsistent procedures. These inaccuracies skew the results and impact AI training.
- Historical bias: Data reflects historical societal biases and inequalities. For instance, historical employment data might show gender pay gaps, and an AI trained on this data could perpetuate that inequality.
Mitigation Strategies
Fortunately, several strategies can mitigate bias in AI algorithms:
-
Data augmentation: Increasing the diversity of datasets is crucial. This involves actively seeking out and incorporating data from underrepresented groups to create a more balanced and representative training set.
-
Algorithmic fairness techniques: Researchers are developing algorithms specifically designed to minimize discriminatory outcomes. These techniques aim to identify and correct for biases within the algorithms themselves.
-
Regular audits and testing: Continuous evaluation of AI systems for bias is essential. Regular audits and rigorous testing, using diverse datasets, help identify and rectify biases before they impact real-world applications.
-
Example: Microsoft's ongoing work in facial recognition technology actively addresses bias through rigorous testing on diverse datasets and the implementation of fairness-aware algorithms. They publicly report on their progress and continually refine their approach.
Promoting Inclusive Design Principles in AI Development
Inclusive design ensures that AI systems are usable and beneficial for everyone, regardless of their abilities, background, or circumstances. This requires a fundamental shift in how AI is developed and deployed.
User-Centered Design for AI
A truly user-centered approach to AI development prioritizes the needs and perspectives of diverse users throughout the entire process. This includes:
- Early user engagement: Involving diverse users in the design process from the outset to understand their requirements and identify potential accessibility challenges.
- Iterative testing and feedback: Continuously testing AI systems with diverse user groups and incorporating their feedback to refine design and functionality.
- Accessibility considerations: Integrating accessibility standards into every stage of development to ensure inclusivity for people with disabilities.
Accessibility and Inclusivity
Designing accessible AI is not merely a matter of compliance; it's about creating technology that empowers everyone. This involves:
-
Multimodal interaction: Designing AI systems that can be used through various modalities, such as voice, gesture, and text, catering to users with different needs.
-
Assistive technology compatibility: Ensuring compatibility with assistive technologies, such as screen readers and alternative input devices.
-
Cognitive accessibility: Designing systems that are easy to understand and use for individuals with cognitive impairments.
-
Example: Microsoft's Seeing AI app utilizes AI to describe the world around visually impaired individuals, demonstrating a commitment to accessibility and inclusive design.
The Role of Transparency and Explainability in Ethical AI
Transparency and explainability are crucial for building trust and accountability in AI systems. Users should understand how AI systems arrive at their decisions.
Understanding AI Decision-Making
Explainable AI (XAI) techniques are essential for providing insights into AI's decision-making processes. This includes:
- Model interpretability: Developing AI models that are inherently more understandable and transparent.
- Decision visualizations: Presenting AI decisions in a clear and concise manner, using visualizations or other intuitive methods.
- User-friendly explanations: Providing users with easy-to-understand explanations of how an AI system reached a particular conclusion.
Accountability and Responsibility
Mechanisms for accountability are vital to ensuring responsible AI development:
-
Clear lines of responsibility: Defining clear roles and responsibilities for developers, organizations, and other stakeholders involved in the development and deployment of AI systems.
-
Auditable systems: Designing AI systems that can be audited to assess their fairness, accuracy, and compliance with ethical guidelines.
-
Transparency in data usage: Clearly communicating how data is collected, used, and protected.
-
Example: Microsoft emphasizes transparency in its AI products by offering insights into how its AI systems function, fostering user understanding and trust.
Collaboration and Partnerships for Ethical AI Development
Ethical AI development requires a collaborative effort involving diverse stakeholders.
Engaging Diverse Stakeholders
This includes:
- Ethicians and social scientists: Their expertise is vital in identifying and addressing ethical challenges related to AI.
- Community groups: Involving representatives from the communities impacted by AI helps ensure that systems are developed in a way that meets their needs and respects their values.
- Policymakers and regulators: Collaboration with policymakers helps shape responsible regulations and guidelines for AI development and deployment.
Industry-Wide Standards and Best Practices
The development and adoption of industry-wide standards and best practices are essential to ensure ethical AI development across the board. This involves:
-
Shared ethical guidelines: Creating and promoting shared ethical guidelines for AI development and use.
-
Best practices for bias mitigation: Establishing and disseminating best practices for identifying and mitigating bias in AI systems.
-
Collaboration on research and development: Fostering collaborative research to address emerging ethical challenges related to AI.
-
Example: Microsoft actively participates in various industry initiatives focused on developing ethical guidelines and best practices for AI, collaborating with other leading technology companies and academic institutions.
Conclusion
Creating ethical and inclusive AI is not merely a technical challenge, but a moral imperative. This hypothetical conversation with Microsoft's design chief underscored the critical role of addressing bias, embracing inclusive design principles, promoting transparency, and fostering collaboration to ensure that AI benefits all of humanity. By prioritizing ethical considerations throughout the AI development lifecycle, we can unlock AI's transformative potential while mitigating its risks. To learn more about building your own ethical and inclusive AI solutions, explore Microsoft's resources on responsible AI development and join the global conversation on shaping a more equitable future powered by AI. The future of AI depends on our collective commitment to ethical and inclusive AI practices.
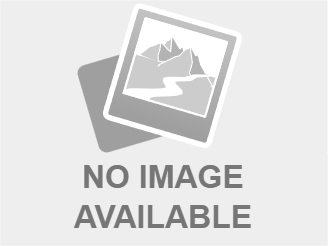
Featured Posts
-
American Battleground Exposing Power And Wealth
Apr 26, 2025 -
Ohio Train Disaster Prolonged Presence Of Toxic Chemicals In Buildings
Apr 26, 2025 -
Elon Musks Private Companies A Potential Side Hustle For Investors
Apr 26, 2025 -
Nfl Draft 2024 First Round Kicks Off In Green Bay
Apr 26, 2025 -
Californias Economic Rise Now The Worlds Fourth Largest Economy
Apr 26, 2025
Latest Posts
-
Crumbach Steps Down Impact On Bsw And The German Coalition
Apr 27, 2025 -
Anti Vaccine Advocate Review Of Autism Vaccine Connection Sparks Outrage Nbc 10 Philadelphia Reports
Apr 27, 2025 -
Hhss Controversial Choice Anti Vaccine Activist To Examine Debunked Autism Vaccine Claims
Apr 27, 2025 -
Anti Vaccine Activists Role In Hhs Autism Vaccine Review Raises Concerns
Apr 27, 2025 -
Anti Vaccine Activist Review Of Autism Vaccine Link Sparks Outrage Nbc Chicago Sources
Apr 27, 2025