Understanding Google's Search AI Training And User Data
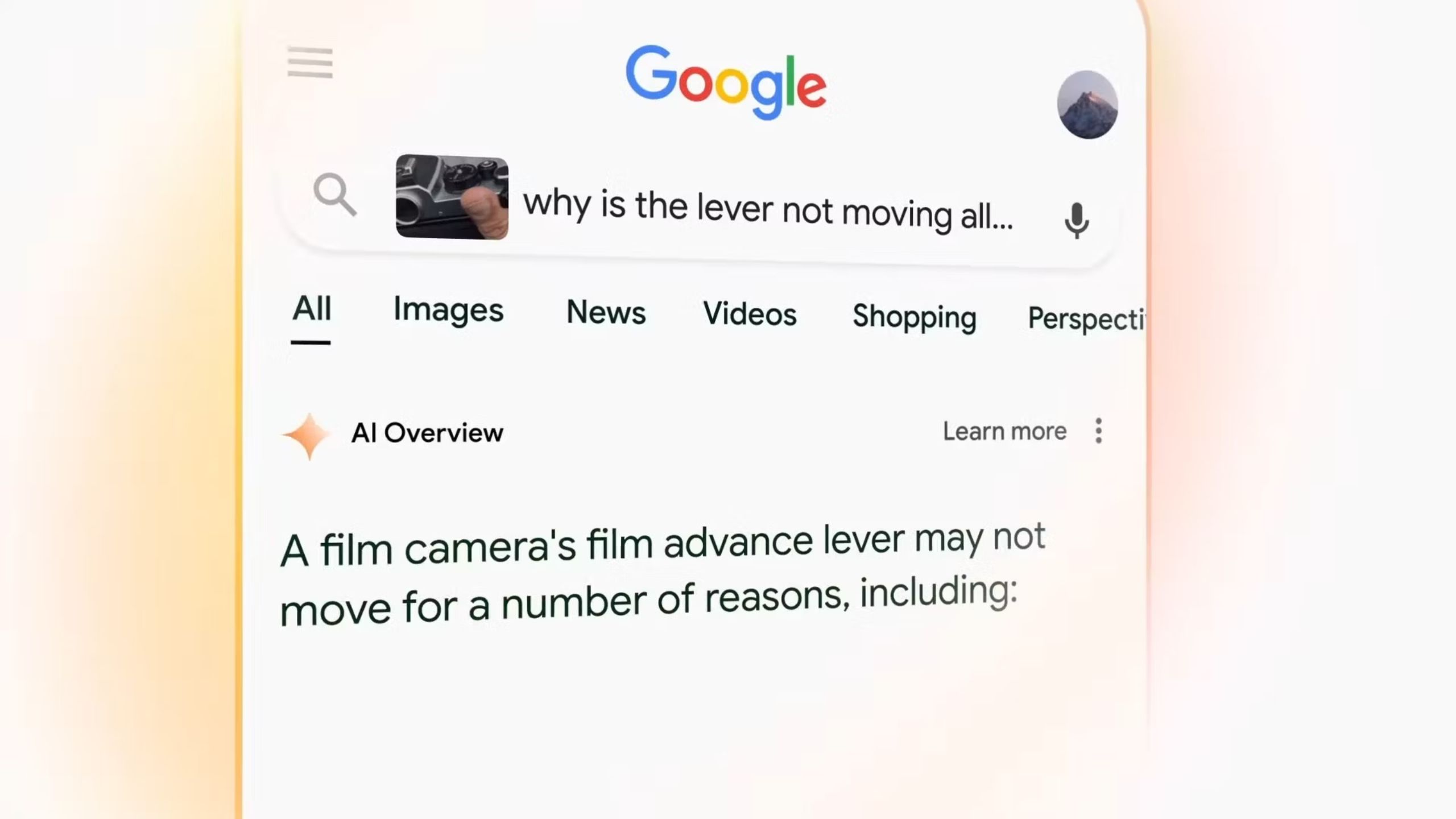
Table of Contents
The Role of User Data in Google Search AI Training
Google's AI-powered search engine wouldn't be nearly as effective without the data it collects from its users. Understanding how this Google AI data collection process works is key to understanding the search engine's capabilities and limitations.
Types of Data Collected
Google gathers a wide array of data to improve its search algorithms. This user data privacy sensitive information includes:
- Search Queries: The actual words and phrases users type into the search bar. This provides invaluable insights into user intent and information needs.
- Clicks: Which search results users click on. This data helps assess the relevance of search results and the effectiveness of the ranking algorithm.
- Location Data: (If permitted) The user's geographical location, which helps tailor results to local businesses and services.
- Browsing History: (If users are logged in) Websites visited, providing context to search queries and improving result personalization.
- Device Information: The type of device (desktop, mobile, tablet) used for the search, influencing the format and presentation of results.
Google employs anonymization and aggregation techniques to protect user privacy. Individual search queries are not usually directly linked to specific users, instead being analyzed in aggregate to identify trends and patterns in search query data.
How Data Shapes Search Algorithm Improvements
User interactions are the lifeblood of Google's search algorithm improvements. Data like click-through rates (CTR), dwell time (how long users stay on a page), and bounce rates (how quickly users leave a page) directly influence the ranking of search results. A high CTR and long dwell time often indicate a relevant and satisfying search result, while a high bounce rate might suggest a poor match.
- Example: If many users click on a specific result for a given query and spend a significant amount of time on the linked page, Google's algorithm will likely raise that result's ranking in the future.
This process leverages reinforcement learning, where the algorithm learns and adapts based on the feedback it receives from user interactions. This iterative process continuously improves the accuracy and relevance of search result relevance and overall AI-powered search experience. Understanding how algorithm ranking works is essential to understanding how Google refines its search results.
Google's AI Training Methodology and Techniques
Google employs a sophisticated blend of machine learning techniques to train its search AI.
Supervised and Unsupervised Learning in Search
Google's search AI uses both supervised and unsupervised learning approaches.
- Supervised Learning: Involves training the algorithm on a labeled dataset, where the correct answers or classifications are already known. For example, Google might train its algorithm on a dataset of search queries and corresponding "best" search results, teaching the AI to associate specific queries with relevant responses.
- Unsupervised Learning: Allows the algorithm to identify patterns and relationships in data without explicit labels. This approach helps identify clusters of similar search queries or discover unexpected correlations in user behavior.
These machine learning algorithms, including various neural networks, are crucial components of the Google search algorithm. The training process is iterative, with constant refinement and improvement based on continuous feedback and new data.
Addressing Bias and Ensuring Fairness
Google acknowledges the challenges of bias in its algorithms and is actively working to mitigate these issues to ensure algorithmic fairness. Bias can creep into algorithms due to skewed data, reflecting societal biases present in the data Google uses.
- Initiatives: Google employs several strategies to address bias, including careful data curation, algorithm design choices that reduce bias amplification, and ongoing audits and evaluations of the search results.
Despite these efforts, achieving complete algorithmic fairness remains a significant challenge in the field of AI, and the ongoing discussion on search engine bias and AI bias underscores the complexity of the issue.
User Privacy and Data Security Concerns Regarding Google Search AI
The use of user data for AI training raises important privacy concerns.
Google's Privacy Policies and User Controls
Google's privacy policies outline how user data is collected, used, and protected. Users can exercise control over their data through features like "My Activity," allowing them to view, manage, and delete their search history and other data.
- [Link to Google Privacy Policy] – Insert a link here.
- [Link to My Activity] – Insert a link here.
Users provide consent for data collection through their acceptance of Google's terms of service and privacy settings.
Potential Risks and Ethical Considerations
While Google emphasizes data security and privacy, potential risks remain. The misuse of user data, even unintentionally, could lead to discriminatory results, privacy breaches, or other ethical concerns. Concerns around AI transparency and accountability also arise in discussions around ethical AI.
- Example: Biased data could lead to search results that unfairly favor certain groups or perspectives.
Responsible AI development and deployment are paramount. This includes transparency in data usage, clear privacy policies, and ongoing efforts to address potential biases and vulnerabilities. The ongoing debate around data privacy risks underscores the need for continuous vigilance and improvement.
Conclusion
The effectiveness of Google Search is undeniably linked to the vast amount of Google Search AI training data it utilizes. This data fuels the sophisticated machine learning algorithms that power the search engine, constantly improving search result relevance and personalization. Google employs various techniques, including supervised and unsupervised learning, to refine its AI models. However, the use of this data raises significant privacy concerns. Google addresses these concerns through privacy policies, user controls, and ongoing efforts to mitigate bias and ensure fairness. Balancing AI innovation with user privacy remains a complex challenge, demanding ongoing scrutiny and careful consideration. We encourage you to explore Google's privacy policies, actively manage your data settings, and stay informed about the ongoing discussion surrounding responsible AI development and Google Search AI training data to make informed decisions about your online privacy.
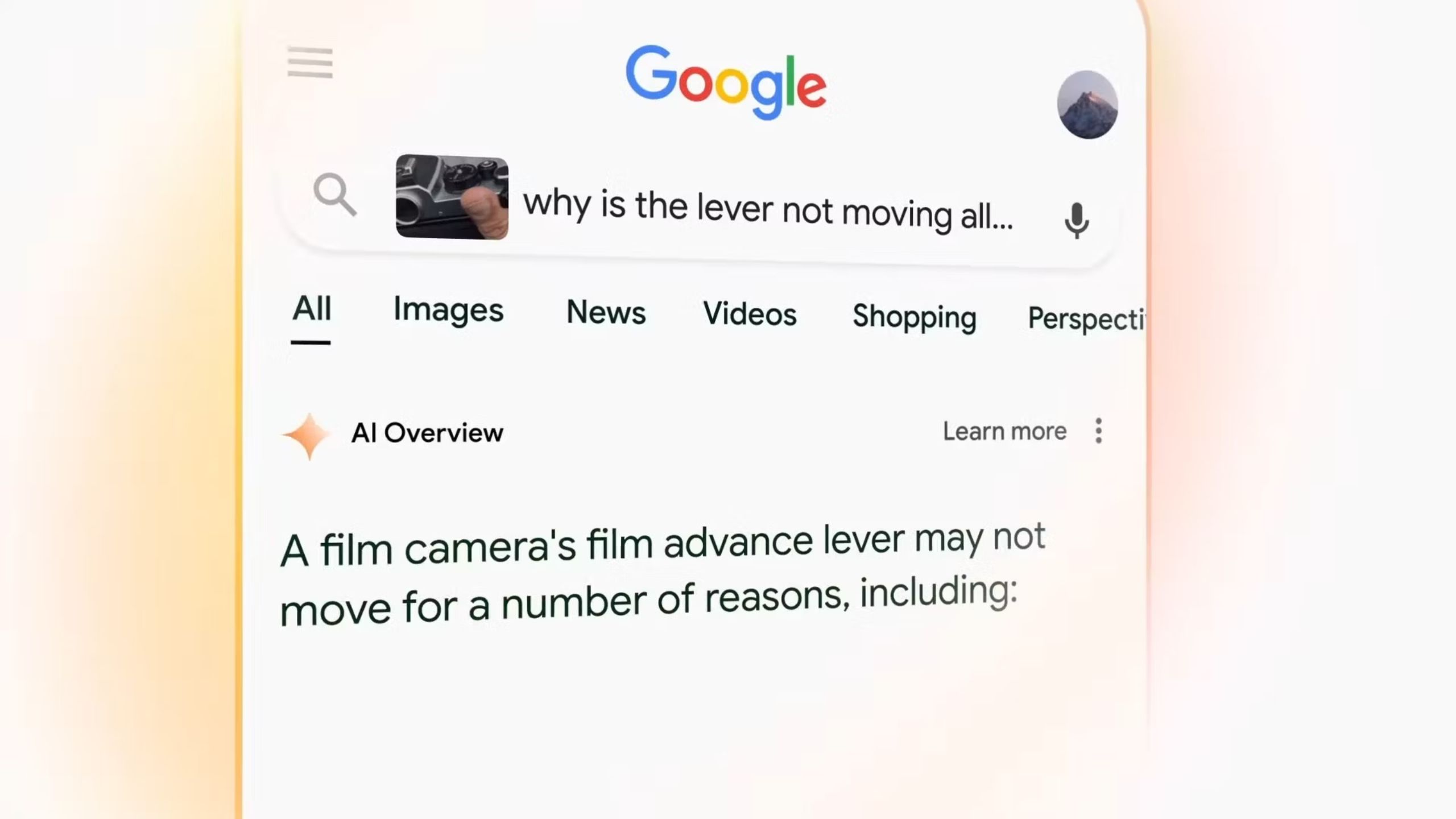
Featured Posts
-
Will Chinas Electric Vehicle Revolution Leave America Behind
May 05, 2025 -
Criticism Mounts After Lizzos Comments On Britney Spears And Janet Jackson
May 05, 2025 -
Marvels Creative Challenges Maintaining Excellence In A Multiverse
May 05, 2025 -
Ai Digest Transforming Repetitive Scatological Data Into Informative Podcasts
May 05, 2025 -
Sheins Stalled London Ipo Us Tariffs Cast A Shadow
May 05, 2025
Latest Posts
-
Lizzos Weight Loss Journey Slimmer Figure Energetic Dance
May 05, 2025 -
Lizzos Show Stopping Curves Take Center Stage In La
May 05, 2025 -
Lizzos Weight Loss Journey The Details Behind The Headlines
May 05, 2025 -
Internet Reacts To Lizzos Striking Weight Transformation
May 05, 2025 -
Lizzos Dramatic Weight Loss Stuns Fans Online
May 05, 2025